Hopefully, you’ve had a chance to read Feedzai’s James Hunt’s insightful conversation with Tom Pilling, Chief Risk Officer at Trust Payments, to discuss Secure and Seamless User Authentication in Payments. Their engaging conversation now continues as the duo outlines ten key fraud prevention tips for businesses.
Read on to learn ten essential fraud prevention strategies for businesses.
1. Embrace the Power of AI
Businesses need advanced fraud prevention tools to protect themselves against AI-driven threats. AI-powered fraud detection systems allow organisations to analyse massive amounts of transaction data in real time, uncovering hidden patterns and red flags that traditional methods might miss. AI-generated alerts should include clear explanations so human analysts can understand the rationale behind potential issues and make informed decisions.
2. Make Human Insights Part of the AI/ML Analysis
AI is incredibly effective at spotting patterns and unusual activity in massive amounts of data. However, AI algorithms can be biassed and need constant monitoring and improvement. That’s why human expertise is still an essential part of AI-based decisioning. A trained human analyst should make the final call on any suspicious financial transaction.
Value-Added Services: Maximizing Revenue through Fraud Prevention
Help Merchants Unlock New Revenue Streams with Value-Added Services Gone are the days when payment processing and hardware were enough. […]
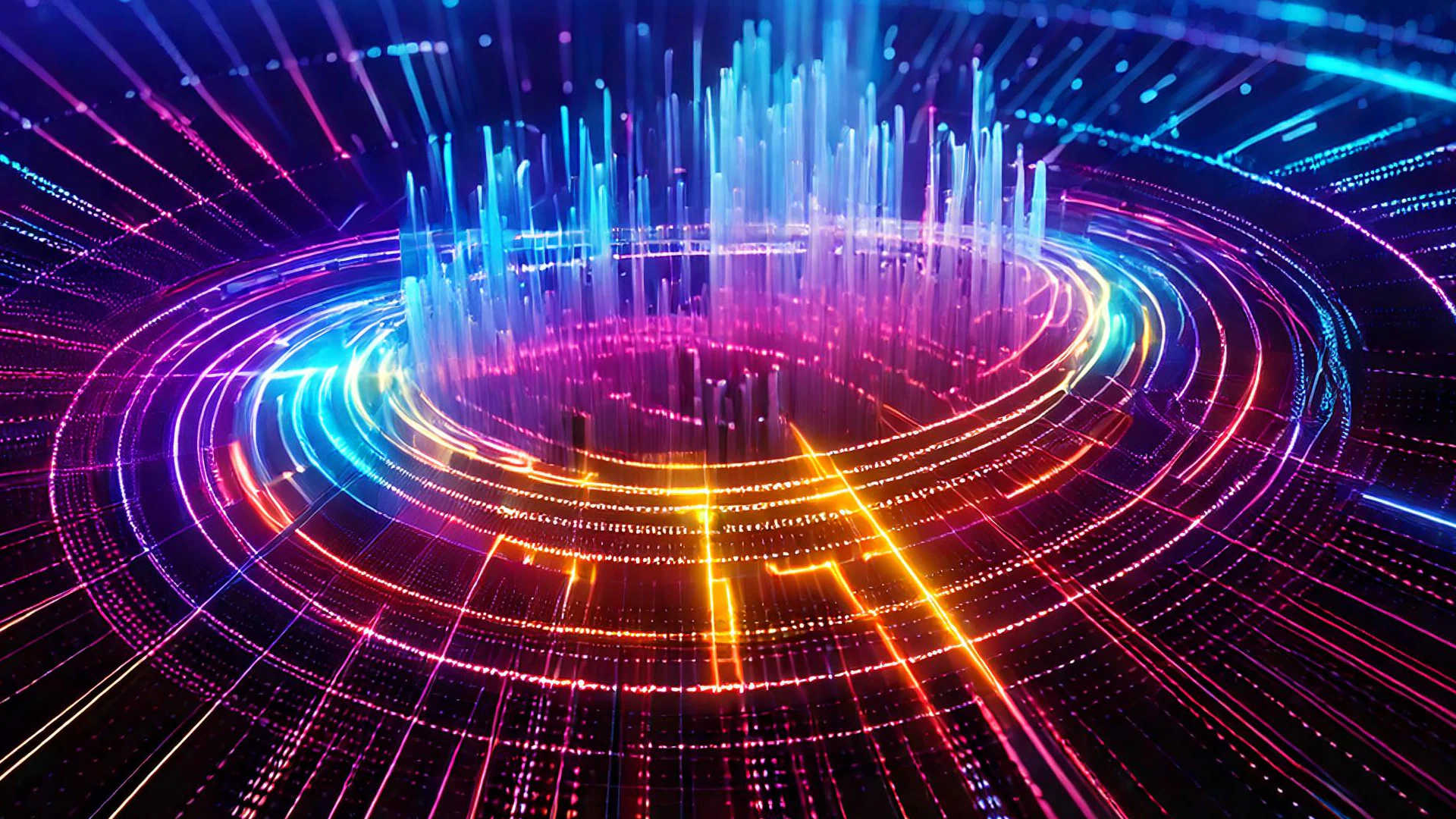
3. Implement Strong Model Governance Frameworks
Strong model governance frameworks are essential for ensuring that AI and machine learning models work as expected, are fair, and don’t cause problems. These frameworks regularly check how well models perform, whether they’re accurate, and whether they’re reliable. Keeping models accurate, fair, and up-to-date to reduce AI risks must be a priority.
4. Collaborate Across the Industry
When it comes to fraud prevention, there’s strength in numbers. Businesses can connect with strong industry players by forging strong alliances and partnerships and joining industry consortiums to stay ahead of the latest fraud threats. These partnerships can grant businesses access to the latest technologies, real-time threat intelligence, and expert insights into how bad actors commit fraud.
5. Use Transactional Data to Turn the Tide Against Fraud
Criminals relentlessly attempt to exploit weak spots in data security. However, businesses can use their transaction data to detect fraud and catch fraudsters. By carefully analysing transaction data, businesses can uncover patterns that indicate suspicious activity. By detecting these patterns, companies can implement safeguards to stop attacks before they happen.
6. Implement Transparent, Intelligent Fraud Detection
Fraud detection and prevention are vital tools to stop criminal activity. But remember, not all fraud detection and prevention systems are the same. Top-performing systems do more than flag suspicious-looking transactions. They also provide clear, transparent explanations for their decisions to allow businesses to take action for themselves.
7. Vigorously Defend Data Privacy
Protecting customer data is non-negotiable. If businesses fail to uphold regulations, including GDPR and PCI DSS, they risk significant fines and damage to their reputations. However, implementing strong security measures does not necessarily require sacrificing customer privacy.
Using device recognition or gauging the speed at which people type allows businesses to identify users without using sensitive information. Measures like these protect customer privacy, access to sensitive personal information, and a business’ financial security.
8. Prioritise the Customer Experience
Security should undoubtedly be a top priority. However, it may require compromising customer experiences. Requiring customers to undergo vigorous, complicated, and frustrating security checks will result in frustrated customers, as well as cart abandonment. Instead, businesses must strike the right balance between strong security and a seamless customer shopping experience.
9. Work with Experts
No business should be forced to tackle fraud threats in isolation. Consider seeking expert help from your payment processor. Partnerships like these can help you understand the changing nature of data privacy and implement the best security measures for your business.
10. Stay Ahead of the Game
Fraud is an ongoing battle. Criminals will always invent new tricks, so businesses must stay ahead.
Read industry news, attend security conferences, and attend webinars to keep up with the latest fraud trends. Share what you learn with your team so everyone can spot suspicious activity. And remember to update your security plans regularly to protect against new threats.
Protect Your Transactions with Fraud Prevention
Businesses need a strong defence plan to effectively combat fraud and earn customer trust. This plan should include implementing the latest technology, partnering with experts, and constantly learning about new fraud threats.
Remember, security is a fluid process, not a one-and-done task. By focusing on these critical strategies and creating a security-conscious workplace, you can build a powerful defence against today’s digital dangers.
All expertise and insights are from human Feedzaians, but we may leverage AI to enhance phrasing or efficiency. Welcome to the future.