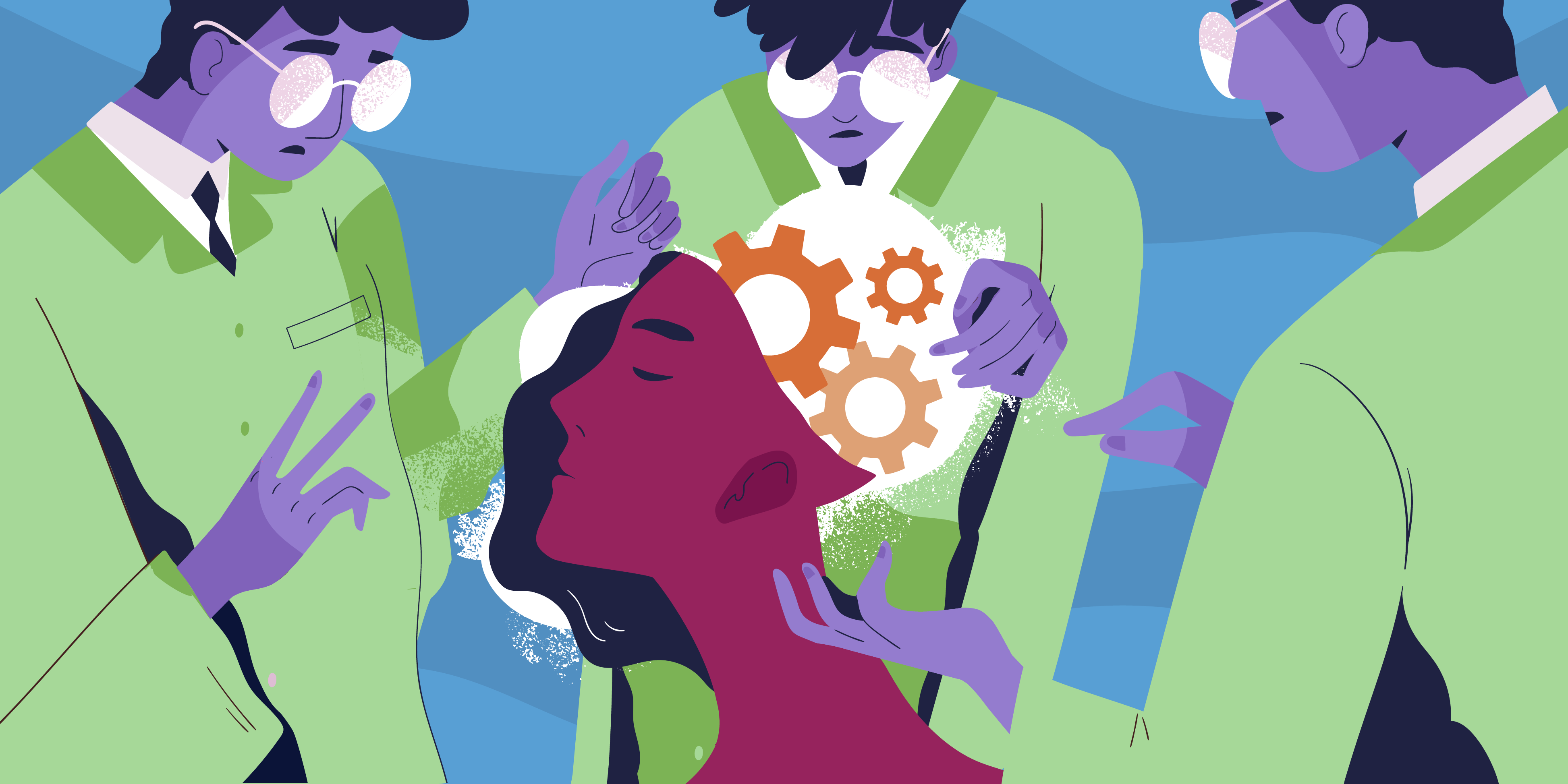
Listen to AI Observability: The Cure for AI Silent Failure (11mins):
Artificial intelligence (AI) systems can learn from vast troves of data to uncover valuable and complex latent patterns. They enable banks to prevent increasingly sophisticated fraud, manage risk and, ultimately, improve results. However, AI systems can suffer from a serious problem: silent failure. In a silent failure, AI systems produce unwanted outcomes. But relevant stakeholders only find out much later that something was wrong. Causes include incorrect or missing data fields from an upstream system or subtle new fraud patterns. AI observability solves silent failure by empowering financial institutions (FIs) to understand how their systems perform in production and quickly adjust any issues in an informed and timely manner.
What is AI Observability?
AI Observability (also known as Observable AI) is a mechanism that continuously delivers insights into how an AI system or machine learning model performs in production over time. It works by collecting data across an AI system, including input data, model predictions, and incoming labels (such as chargebacks) to create a feedback loop for relevant stakeholders.
Stakeholders rely on these insights to perform a 360º assessment of whether a production system acts consistently and behaves as intended across a wide range of criteria. If not, stakeholders can proactively collaborate to introduce any necessary improvements or adjustments. Thus, observability fosters human oversight, accountability, and adaptability. As the saying goes, “you can’t manage what you can’t measure.”
AI observability is a key enabler for FIs’ Responsible AI initiatives, providing greater transparency and visibility into how the system impacts end-users over time in dynamic production environments rather than static offline settings.
Why FIs Should Care About AI Silent Failure
AI systems predict whether incoming transactions are fraudulent or legitimate based on the transaction characteristics and a combination of model scores and hard-coded rules. However, weeks later, while reviewing KPIs or during a manual ad-hoc system check-up, the bank learns that the fraud losses increased due to a spike in uncaught fraud. In other words, a large share of fraudulent transactions went undetected.
Note that the system was fully operational during this period. The issue at hand relates to the quality of the system’s outcomes. Hence, the bank learns their system failed silently.
There are several reasons why system outcomes can differ from the expected behavior. Perhaps the data changed over time, either suddenly or gradually. Or maybe fraudsters discovered a new tactic that the system failed to detect. Alternatively, even if the system’s overall behavior meets expectations, problems may occur for specific segments of clients or locations and, therefore, be unobservable at the top level.
Whatever the reason, without ongoing insights into how the AI model performs in production, the bank suffers higher fraud losses. Critically, the problem becomes evident after the system produces unwanted outcomes. By then, data science teams must go back to the drawing board to learn why the system failed and fix it, often with insufficient data on what is happening live.
4 Stages of a Feedback Loop
Silent failure can be costly because stakeholders only learn of it after it’s too late. It can impact the quality of services provided to customers and result in financial losses, compliance fines, and reputational damage. AI observability addresses this by introducing ongoing feedback loops that relay important information about the health and quality of a system’s data, model, and decisions. Here’s how feedback loops work.
Step 1: Monitoring
The first step is to collect the necessary data to monitor how an AI system behaves over time. Data collection should cover a diversity of signals, including the system’s inputs (e.g., raw data), outputs (e.g., the system’s decisions), and all relevant intermediate steps (e.g., feature engineering, model scores, and rule triggers).
Step 2: Metrification
The next step is to detect unexpected changes in the system’s behavior. AI Observability requires dedicated metrics that express how the system’s behavior shifts over weeks, months, or longer windows once the model is in production. The combination of metrics should provide a 360º view of the system at an appropriate granularity level.
Step 3: Alarmistics
Once significant deviations are detected, it is critical to alert stakeholders. A typical implementation proactively introduces alarmistics so that significant deviations (e.g., changes in the data, emerging patterns) trigger alarms to the relevant teams.
Step 4: Explanations
Finally, the feedback loop must produce interpretable explanations for metrics and alarms. Usability is a crucial component of human oversight and essential to accelerating corrective actions. Proper visualization techniques make it easy to understand and address the issues at hand. Stakeholders can continuously review system indicators and determine the best course of action.
Why is AI Observability Important?
Here are some key reasons why AI observability should be a top consideration in financial services.
Detect new fraud patterns faster
Unfortunately, the financial services sector attracts hostile actors looking to bypass financial crime prevention systems. Once AI-based fraud detection systems notice a fraud pattern, fraudsters start testing new ones. AI Observability is a natural fit for such a dynamic environment because it identifies changes in the data and the system’s response. Continuously monitoring an AI system is the first step towards adaptability and ensuring high performance over time.
A notable example of shifting patterns happened when both licit and illicit actors adjusted their behavior because of the COVID-19 pandemic. Without proper AI Observability, AI systems can be opaque, making it hard to understand the impact of these changes.
Catch more bugs
AI systems typically interact with several other systems. Therefore, interface changes, unforeseen edge cases, and other problems are commonplace. Even if these problems don’t undermine the system’s prediction capabilities, they can affect their quality. If these bugs impact the AI system, AI Observability can quickly detect the problem and alert stakeholders.
Close the fraud label gap
It is typical to measure a model’s performance by comparing its predictions to the eventual outcomes, known as the labels. The trouble with this approach is that fraud labels are not immediately clear. For example, FIs don’t realize some transactions are fraudulent until after a customer reports it to their bank. Banks will eventually conduct a review and retroactively label these transactions as fraud. This means the model performance evaluation needs to consider known legitimate transactions, known fraudulent transactions, and transactions for which the label is unknown. AI Observability for FIs requires techniques for ongoing monitoring that can close the label gap.
Fulfill Responsible AI expectations
Banks and FIs that implement AI should also commit to ensuring that they follow responsible AI principles. Human biases and systemic social issues can infiltrate AI systems even unintentionally. The result can be unfair financial decisions that disproportionately impact certain groups of people based on their race, gender, age, or socioeconomic status. AI Observability enables FIs to understand how their systems treat people over time. Stakeholders can quickly respond to AI bias patterns before they balloon into much larger issues. This is essential in addressing the problems of Responsible AI once a model is deployed.
Unexpected developments such as model degradation, emerging fraud patterns, or shifts in the data can throw off an AI system’s performance. AI observability gives stakeholders visibility into whether AI systems are behaving as expected or need to be adjusted, enabling proactive maintenance.
By implementing Responsible AI principles, FIs can make sure their AI aligns with their ethical values. But what are the risks and benefits involved? Watch our on-demand webinar Responsible AI in Financial Crime Prevention to learn how bias gets into AI systems and how to mitigate it.
Share this article:
Related Posts
0 Comments11 Minutes
#MyAI: Customer Choice and Consent in the Generative AI Age
From chatbots to call centers to loan applications and beyond, artificial intelligence…
0 Comments11 Minutes
Feedzai Fraud Frontlines: Cloud Migration Done Right
We’re on a mission to help banks and financial institutions shield their customers from…
0 Comments6 Minutes
How Feedzai Supports Mental Health / Menstruation at Work
At Feedzai, we have a reputation for being risk-takers and innovators. In our product, in…