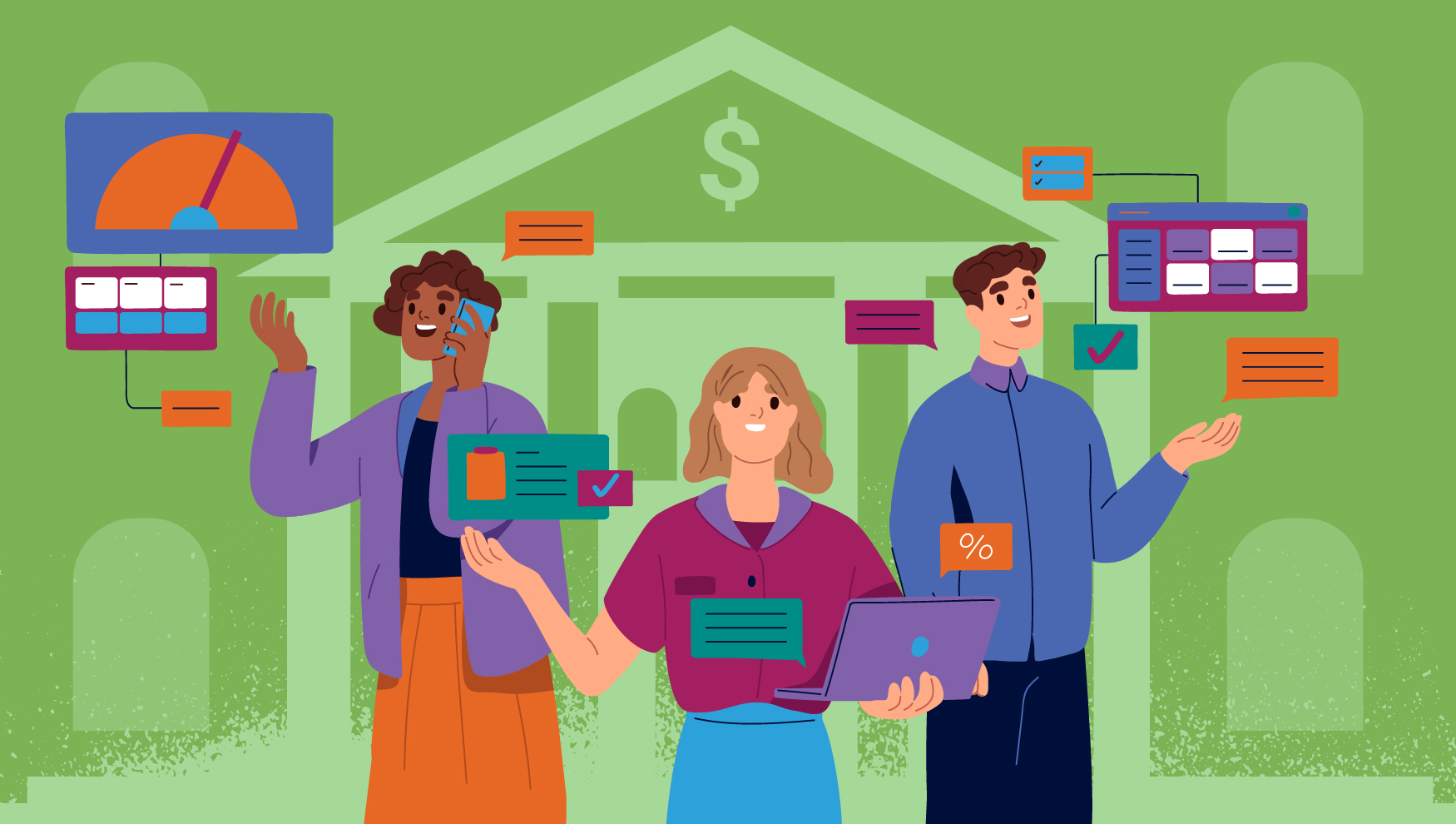
There have been so many artificial intelligence-related developments lately that it’s hard to keep track of them all. From conversational tools like ChatGPT, Bard, and Microsoft’s Bing program to visual programs like Dall-E and Midjourney. And that’s just a few that come to mind. Here at Feedzai, AI has always been part of our DNA. That’s why we’re excited to share how AI can enhance the anti-money laundering (AML) transaction monitoring process.
2 Key Areas Where AI Boosts AML Monitoring
There are multiple use cases where banks can use AI to enhance their AML transaction monitoring capabilities. These use cases typically fall into two distinct categories: detection and operations.
The key principle of detection comes down to a simple concept: keeping your regulators happy. AI can reduce the risk of your financial institution becoming exposed to financial crime. On the operations side, AI can help compliance analysts use their time more effectively, especially when it comes to opening suspicious activity reports (SARs).
Let’s dive further into how AI enhances each use case.
Part 1. How AI Boosts AML Detection in 3 Steps
It’s tempting for banks to think about AI and machine learning as a one-and-done task. After all, once you build a model and train a model, it’s easy to think that the hard work is behind you. However, at Feedzai, we assure you that this is just the beginning of your bank’s machine learning journey. Implementing AI for AML detection requires a vision and execution of several important steps.
We advise our customers to follow a three-step process for their machine learning and AI journeys.
1. Start by Supplementing Existing AML Rules with ML
Start improving AML operations with existing rules by getting input from your analysts. These rules will generate alerts and the alerts will prompt action from your operations team. This includes filing SARs or escalating alerts. Collecting analyst feedback on the performance of rules and alerts is an essential component of a successful ML system.
2. Add Supervised Machine Learning to Your Rules
After supporting your existing rules with machine learning, you can then implement supervised machine learning. This involves training supervised models based on your analysts’ feedback signals. Like any other machine learning solution, your supervised models will require training until you are confident in their performance.
For example, is the model capable of reducing the number of false positives after learning the patterns from the rules? If rules are defined well, the models will be able to see new risky patterns in a holistic way. As a result, analysts will have very high confidence when they trigger alerts. Even if the first or second rule triggers an alert, the model will be able to predict with 99% accuracy that it’s a false positive. Once you gain confidence in the model, you can use it to reduce false positive alerts and generate more insightful and useful ones.
3. Implement Unsupervised Machine Learning
In the third stage, your bank can implement unsupervised machine learning models. There are several activities that may look suspicious to your bank, but analysts will not know exactly what to look for. In other words, what are the known unknowns? Designing rules to uncover known unknowns is extremely challenging.
For example, imagine that you get 10 alerts pointing to strange activity each week. It’s not necessarily suspicious, but it definitely is strange and unexpected. The model has never encountered a situation like this before. Because it’s an unknown event, the model can trigger an alert for further review. Once the review is complete, you can incorporate the feedback back into your rules or your supervised machine learning model.
At the end of the day, you’ll end up with a very mature system combining the rules of a static AI system with supervised machine learning, and unsupervised machine learning, all in one system. With this combination, your bank will become much more agile in its AML transaction monitoring program.
Part 2. The Operational Side of AML Transaction Monitoring
Beyond detection, banks should also focus on upgrading their operations for AML systems. Enhancing operations can free up how analysts spend their time. Improving operations automates much of the analysts’ workflow and allows them to focus on what’s most important to them instead.
Analysts typically have to work with lots of different systems that require a heavy manual lift. They often need to copy information from multiple sources and paste it into a big Excel spreadsheet. From there, they need to perform a series of manual queries in the document. This process is both error-prone, highly time-consuming, and inconvenient.
This disparate arrangement makes it difficult for analysts to do their jobs efficiently. What would make it easier is to have all the data in one place instead of being forced to consolidate and review hundreds of transactions at a time. This includes the transaction monitoring system, reference data, and relevant enrichments. If all of these elements are centralized in one place, analysts can easily access the important information they need. When an alert is triggered, the system can present that information in the most useful format for the analyst.
Concentrating data in a centralized location can spare analysts a cumbersome review process. They can instead review transactions in a more efficient way, often with the help of visualization tools. From there, analysts can look at network information with graph analytics and determine where money is moving. In other words, analysts can “follow the money” more easily.
Analysts shouldn’t have to waste time connecting pieces of information as if they were solving a jigsaw puzzle. And they shouldn’t have to manually review their work. By improving operations, analysts can spend more time studying the pattern right in front of them. From there, they can make an assessment if the pattern is suspicious or not, if it needs an escalation, or if they should file a SAR.
AI has transformed significantly in the past year alone. Now is the time for banks to ask themselves how to use this technology to upgrade AML operations and stop financial crime.
Share this article:
Related Posts
0 Comments5 Minutes
Spotlight on Denmark: Fraud and Financial Crime Insights from ‘Den sorte svane’
The recent documentary mini-series "Den sorte svane" has sent shockwaves through Danish…
0 Comments9 Minutes
Enhancing AML Transparency with Smarter Data
Doesn’t it seem like new financial threats crop up in the blink of an eye? That’s why…
0 Comments10 Minutes
Enhancing Anti-money Laundering Systems Architecture
A speaker at a financial crime conference I recently attended summed up the problem with…