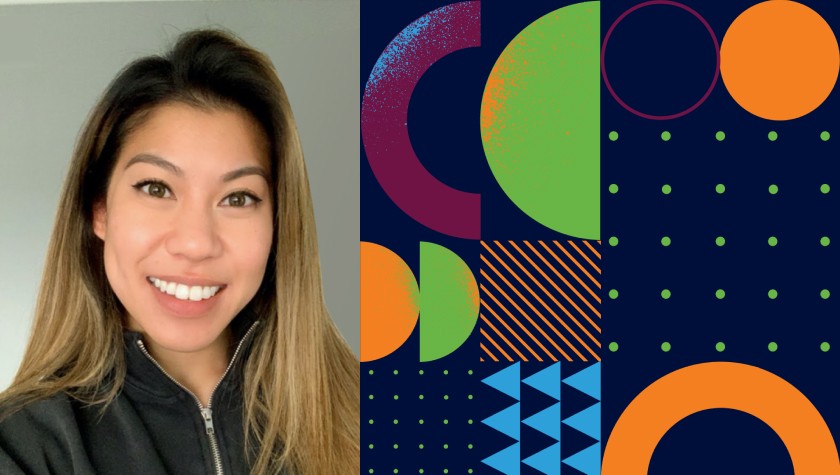
Artificial intelligence (AI) and machine learning are pivotal in helping banks and institutions stay ahead of fraud and financial crime tactics. However, advanced technologies come with their own set of challenges, especially when it comes to model risk governance, a comprehensive and structured approach to managing the risks that arise from the development, deployment, and continuous operation of quantitative AI models.
Learn the critical challenges with current AI model risk governance frameworks and how Feedzai is making a difference.
The Challenges with Current AI Model Risk Governance Frameworks
Many banks face two key challenges regarding AI model risk governance frameworks.
1. Self-Learning and Evolving Models
AI models are not static entities. They self-learn and evolve after exposure to real-world scenarios.
This dynamic nature can be a double-edged sword. On the one hand, it helps catch unexpected anomalies that traditional systems might miss. But on the other hand, it poses a challenge for fraud teams. Banks must ensure that these models continue to produce meaningful results.
2. Understanding Supervised and Unsupervised Models
Two primary types of machine learning models come into play here: supervised and unsupervised.
- Supervised machine learning uses training data with labels, identifying “good” or “bad” examples. The model learns to classify new examples based on patterns found in the training data.
- Unsupervised machine learning takes a more autonomous approach because it is not trained on labeled data. It identifies anomalies based on clusters of data that it deems similar. This makes it a powerful tool for uncovering unexpected fraud patterns.
While the advantage of unsupervised models is clear, it is crucial to maintain vigilant oversight to guarantee their continued efficacy in real-world applications.
2. Regulatory Expectations for Governance
Many jurisdictions, such as the US Office of the Comptroller of the Currency (OCC), mandate the documentation of the entire process involved in creating and maintaining a model that affects individuals’ financial decisions. This documentation is a crucial step in ensuring fairness and accountability in using models. However, there are several challenges to overcome:
1. Domain Expertise is Critical (and Time-Consuming)
Effective model governance typically requires a dedicated team who is hands-on with the model development and monitoring process. This team should also clearly and explicitly communicate how they use the results.
This process isn’t necessarily flashy because this governance process doesn’t actively stop criminals. But it’s crucial to always monitor and tune models, as well as demonstrate the validity of the decisions your financial institution produces.
Furthermore, it’s detrimental if it’s done incorrectly. Imagine if your credit bureau cannot prove its methodology for generating your credit score.
End-to-end documentation of data sources, the intended purpose, development, training, and results of all models is a time-consuming process. A team needs to sit down and type out a multi-page report detailing this process with tables, graphs, and charts to demonstrate the model’s purpose and effectiveness. Some regulatory agencies require this to be done on a semi-annual basis.
2. New AI Techniques Carry Risks
The advent of generative AI, or GenAI, introduces a new set of risks that go beyond the model itself. Transparency is key, and it’s vital for building trust in the decisions made by these models. It’s critical to understand and document where the data originates.
Consider this scenario: If the data sources are not transparent, how can banks trust the responses or decisions that AI models provide? Or explain it to regulators? For example, if models draw from biased data sources, questions about fairness and reliability will arise. When AI models impact people’s lives by determining whether they can open a savings account or get a credit card, transparency and reliability become paramount.
The Biden administration recently issued the first-ever executive order on artificial intelligence’s societal impact. The order aims to ensure AI is implemented safely, prepare agencies for AI’s growth, and mandate transparency from model developers. In other words, expect model transparency to be required as new AI regulations take shape.
How Feedzai Stands Apart
Feedzai understands these challenges and has crafted solutions that set it apart in the field of AI model risk governance.
1. Automatic Model Monitoring
Feedzai’s proactive monitoring system automatically detects changes in models often unseen by the human eye. This process begins with feature engineering and the automatic selection of the best features for the model. As the model produces results, it’s crucial to ensure that it still performs as intended. Feedzai streamlines this aspect, saving time and resources.
2. Automatic Feature Monitoring
Data drift is a significant concern in AI model governance. Feedzai offers automated alarms and data drift detection by monitoring the distribution of data features. It measures data stability by comparing feature distributions over time, thus providing insights into potential issues.
A real-world example from a bank in EMEA found broken fields and data drift when comparing training data with production data. Intuitive visuals explain observed shifts in the data, simplifying the decision-making process.
3. Automatic Model Governance Reports
Feedzai automatically generates standard Model Governance Reports with all the relevant information and numbers, such as data sources, features used by the model, detection performance, and a bias audit. The system captures any edits or changes made to the model, automatically documenting them within the system. Banks can easily pull these details into the report, saving considerable time and effort.
Why Choose Feedzai?
Feedzai delivers a different AI model risk governance experience for banks. This experience features two important benefits.
- Built-in Value: Time is valuable for any organization. Banks can either handle all these tasks manually or build similar systems themselves. This can be a resource-intensive and time-consuming endeavor.
- Time and Cost Savings: Banks can also reduce the time and effort required for model governance. The system does the heavy lifting, allowing institutions to make and document changes efficiently.
These benefits cater to both bigger and smaller banks. Larger organizations will see report preparation times reduced from two weeks to a few days. This enhances efficiency and allows for quicker response to evolving fraud patterns. Meanwhile, for smaller banks, which may not have robust model governance systems, the system helps reduce risks and boost capabilities.
Feedzai delivers automated monitoring, feature analysis, and report generation that ultimately saves banks time, money, and resources. Feedzai ensures that AI models provide effective results and are adaptable in the shifting landscape of financial crime detection.
Share this article:
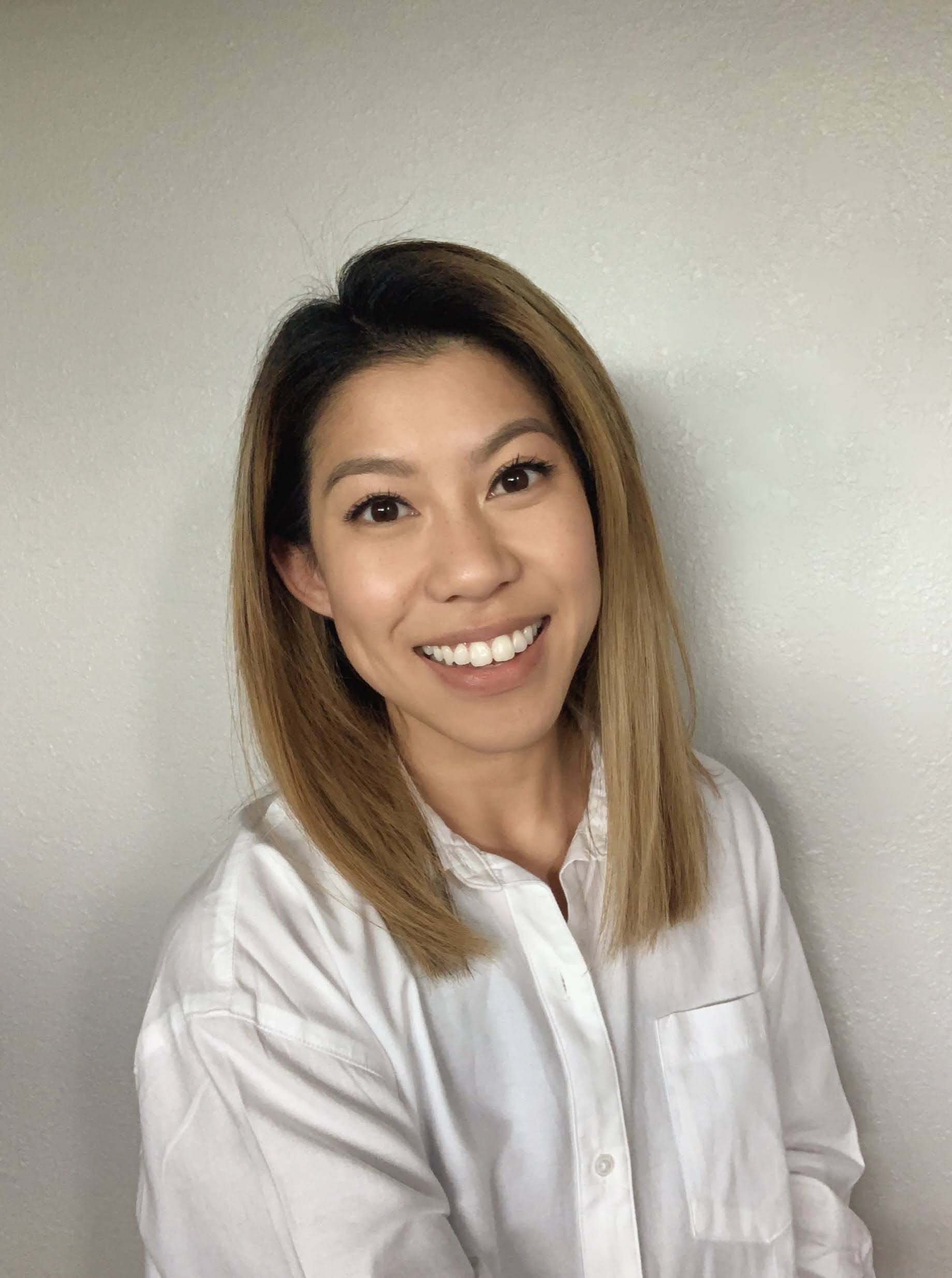
Tiffany Ha
Tiffany Ha is a Sr. Product Marketing Manager at Feedzai. She has spent the last eight years in the fraud and financial crime prevention space. Financial crime trends and tactics are always evolving, so the accompanying technology must stay ahead of the curve. She loves connecting the dots between technology and market challenges to help financial institutions achieve their goals.
Related Posts
0 Comments10 Minutes
Boost the ESG Social Pillar with Responsible AI
Tackling fraud and financial crime demands more than traditional methods; it requires the…
0 Comments14 Minutes
What Recent AI Regulation Proposals Get Right
In a groundbreaking development, 28 nations, led by the UK and joined by the US, EU, and…
0 Comments12 Minutes
Built-in Responsible AI: How Banks Can Tackle AI Bias
Many bank customers know that banks use artificial intelligence (AI) to make decisions.…