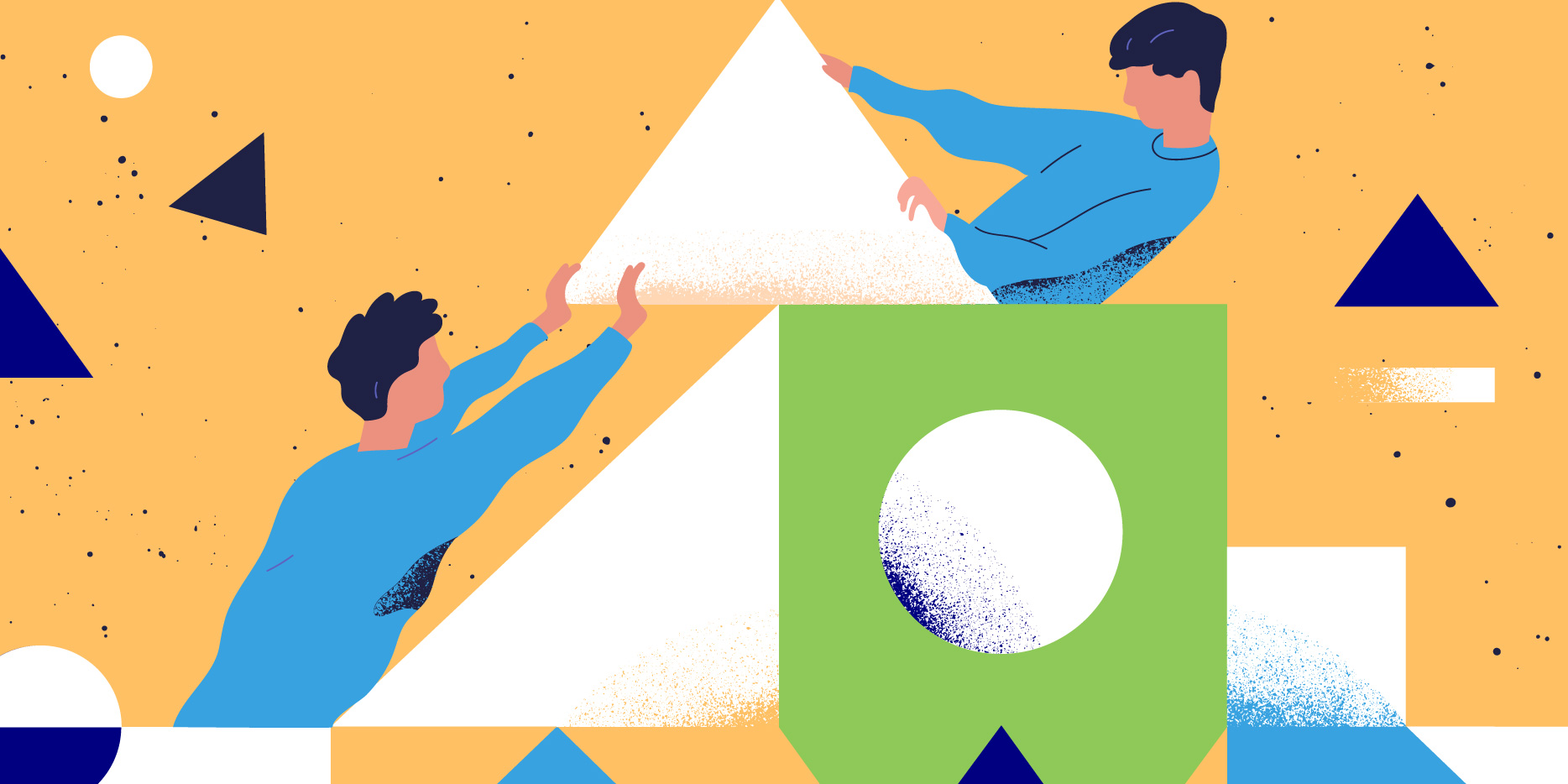
Fellow data scientists, have you ever wondered what your biggest data science motivation is? Is it to hit that ever-elusive target right before the submission deadline? Or is it something more along the lines of implementing and testing that fancy but obscure algorithm you saw on KDD?
Data science in the wild
More often than not, you will find yourself driven by these seemingly opposite motivations, especially during the application process for your first data science (DS) job after graduation. Consulting companies usually focus on delivering something data science-y that meets well-defined criteria. On the other hand, other companies have data scientists that mostly concentrate on applying (or beating!) state-of-the-art algorithms used in their industries.
So, the question is, can we aim to do both? Can we deliver results and pave the way forward?
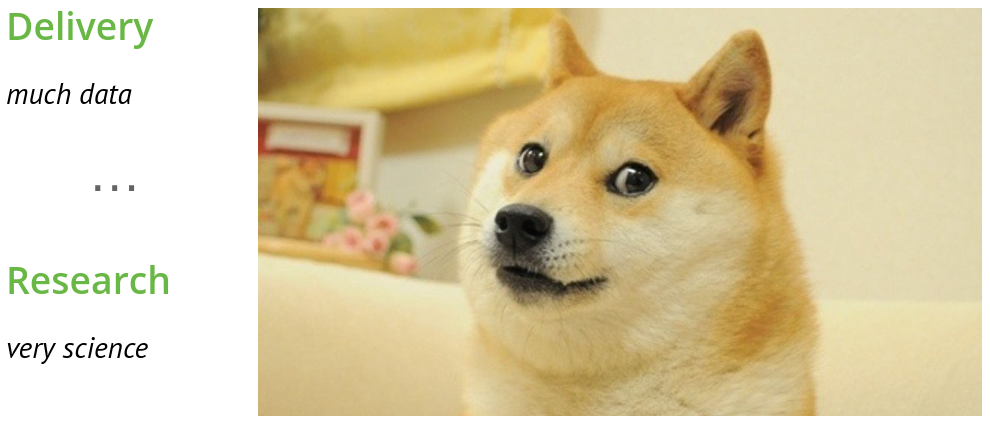
That’s where data scientists come in.
There are different types of data scientists. It’s always fun to reflect on how their responsibilities are viewed from different angles:
Delivery data scientist
- What customers think I do: solve all the problems, like a hero, while the clock is ticking.
- What other DS teams think I do: attack problems by brute-forcing more features and models.
- What I actually do: reply to customer emails about weird data issues.
Research data scientist
- What customers think I do: build algorithms that will take over the world and make all jobs obsolete.
- What other DS teams think I do: scribble advanced math completely detached from actual problems.
- What I actually do:
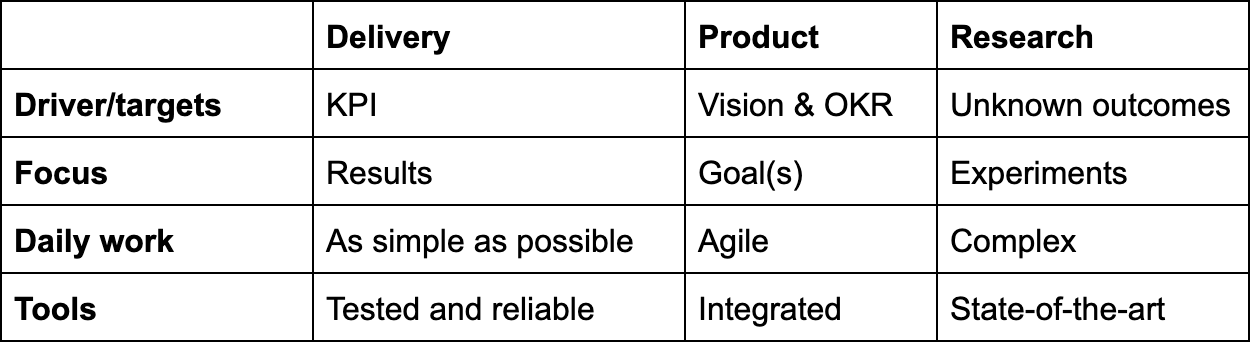
Product data scientist
-
- What customers think I do: provide them with a polished product.
- What other DS teams think I do: put on a façade.
- What I actually do: spend a substantial amount of time in front of a whiteboard with post-it notes, figuring out priorities.
It seems we tend to categorize data scientists based on our perception of their skillset. Just search for job postings that call for any of these data scientist roles, and you’ll see what I mean. (I must say it made me chuckle when I noticed that “perform A/B testing” was the skill most commonly asked of “product data scientists!” ?) However, what actually distinguishes different data scientists from one another is what they do — not how they do it.
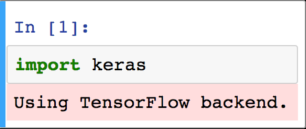
The key lies in the function
These three kinds of DS roles almost look the same from a technical standpoint. In fact, data scientists in these positions share nearly identical skillsets and carry out similar tasks. When we zoom out and see how these various data scientists contribute to companies, however, the contrasts rise to the surface: there may be technical overlap between different roles, but there are some significant functional differences.
So, what do all these observations tell you? If you feel that there are now more questions than answers, rest assured. Everything is going as planned. In the next installment of this 3-part blog series, we will discuss what the focus of DS should be on a product team — practical examples included!
Share this article:
Related Posts
0 Comments10 Minutes
Boost the ESG Social Pillar with Responsible AI
Tackling fraud and financial crime demands more than traditional methods; it requires the…
0 Comments8 Minutes
Enhancing AI Model Risk Governance with Feedzai
Artificial intelligence (AI) and machine learning are pivotal in helping banks and…
0 Comments14 Minutes
What Recent AI Regulation Proposals Get Right
In a groundbreaking development, 28 nations, led by the UK and joined by the US, EU, and…