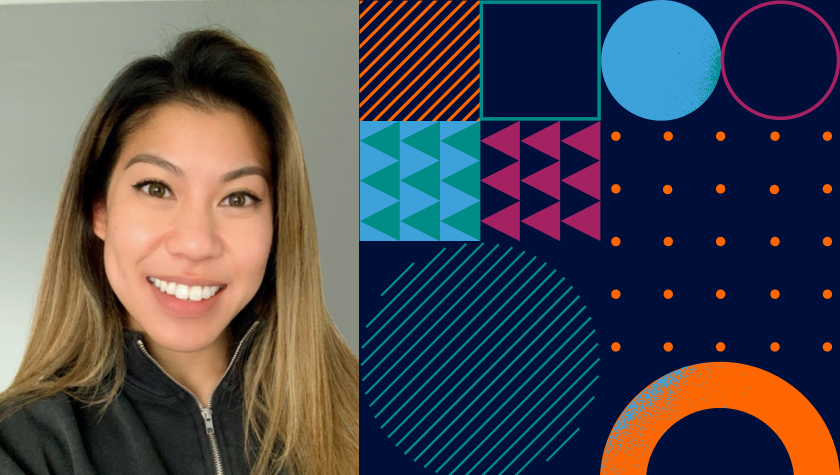
Many bank customers know that banks use artificial intelligence (AI) to make decisions. Yet, they also want their bank to treat them fairly and without bias. With built-in Responsible AI, banks can be both fair and efficient in their AI decisions.
Some people think that to make AI fair, it might become less efficient. But at Feedzai, experts in Responsible AI, we believe that’s not the case. In this article, we’ll show how banks can use Responsible AI to be both fair and effective. Plus, we’ll discuss how it lets banks choose the best models for them.
What is Built-in Responsible AI?
Responsible AI is a framework that ensures decisions reached by an AI or machine learning model are fair, transparent, and respectful of people’s privacy. The framework also empowers financial institutions with explainability, reliability, and human-in-the-loop (HITL) design that offers guardrails for AI risks. Built-in Responsible AI, meanwhile, offers banks a seamless pathway to implement fair AI and machine learning policies and procedures without compromising on their system’s performance. Banks are presented with options that offer fairer decisioning. However, banks are not obligated to select these options and can choose the framework that works best for their purposes.
Biases can arise at different stages of model building or training. As a model self-learns in production, it may develop biases that were not intended by the developers of the model. Beyond that, bias can even creep in from the bank’s internal rules and the humans responsible for making decisions over customers’ financial well-being. This means banks may deny important financial services, including access to bank accounts, credit cards, bill payments, or approval of loans to qualified individuals. This is not deliberate, but because a machine learning model found they are the “wrong” gender or come from a “high-risk” community, they find themselves unfairly financially excluded.
Political leanings can also influence a bank’s decision-making. In the UK, for example, the government is investigating whether some customers are being “blacklisted” from critical financial services over their political views.
Every bank is committed to giving its customers the best possible service it can provide. At the same time, banks want to treat every customer fairly and compassionately. As banks rely increasingly on artificial intelligence and machine learning for faster decision-making, they must trust their models to meet these priorities.
Why Built-in Responsible AI is Critical for Banks
As AI technology becomes increasingly prevalent in financial services, banks will need to stay vigilant in monitoring for bias. With new AI-based technologies gaining prominence, this is a mission-critical mindset.
Case in point, a recent study on biases in generative AI showed that the text-to-image model thought that “high-paying” jobs, like “lawyer” or “judge,” are occupied by lighter-skin males, while prompts like “fast-food worker” and “social worker” are occupied by darker-skin females. Unfortunately, in this example, AI is more biased than reality. For the keyword “judge,” the text-to-image model generated only 3% of images as women. In reality, 34% of US judges are women. This exemplifies the considerable risks of unintentional bias and discrimination in AI, which negatively impact operations, public perceptions, and customers’ lives.
Consumers are increasingly aware that AI is used to generate answers on any topic and ultimately help people make informed decisions faster. If they believe they were treated unfairly by their bank, they may ask to see and better understand the bank’s decision-making process.
The False Choice Between Model Fairness and Performance
Unfortunately, banks are often convinced that they must trade off fraud detection performance for fairness and vice versa optimize their models for maximum efficiency and performance over fairness. Without an accurate way to measure both. As a result, many banks are forced to prioritize performance to boost their bottom lines. Model fairness and Responsible AI get treated as “nice to have” agenda items. But neglecting to prioritize model fairness allows biases to creep into a bank’s model, even if it’s never intended.
To put it mildly, this is a problematic approach for banks. Not only is it a false choice, but it’s also a risky one that can have harmful consequences for banks if they ignore biases in their models for too long. If too many groups of customers believe they were denied services because of their age, gender, race, zip code, or other socio-economic factors, it can create a significant public relations headache for the bank and possibly litigation.
How Feedzai Delivers Built-in Responsible AI for Banks
Feedzai has worked for years to find a way to avoid having to make a choice between model performance and model fairness. As pioneers in Responsible AI in the fraud and financial crime prevention space, we’re committed to changing this narrative.
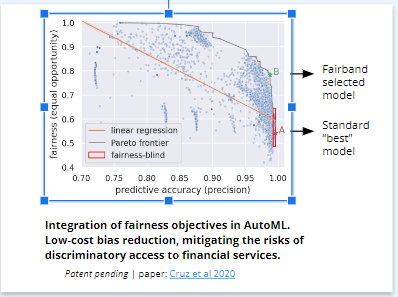
Our culture of Responsible AI comes from the top down with a team of passionate leaders dedicated to doing the right thing for customers. It’s an honor to have industry experts like IDC recognize Feedzai for our work.
How Built-in Responsible AI Works
Feedzai’s Built-in Responsible AI tools provide financial institutions with the tools they need to tackle model bias before it gets out of hand. These tools enable banks to quantify bias, automatically identify fairer models, and optimize models for both fairness and performance.
Here are Feedzai’s key tools for built-in Responsible AI.
Bias Audit Notebook
A bank’s first obligation is to assess and measure the bias of its models. Feedzai’s built-in Responsible AI tools provide a bias audit notebook where banks can visualize and quantify the level of any bias they uncover. Conducting a risk audit empowers banks to understand what type of attributes put them at risk of creating bias. The bias audit notebook allows you to incorporate information in the model’s selection process by selecting algorithms that maximize fairness. This enables banks to uncover and fix bias before it becomes a problem or a threat to the bank’s reputation.
FairAutoML (Feedzai Fairband)
Banks can also automate the model selection process using Feedzai Fairband. Fairband is an award-winning automated machine learning (AutoML) algorithm that can quickly identify less biased models that require no additional training to implement. This means financial institutions can quickly deploy the fairest models available without compromising performance. While Fairband adjusts the hyperparameter optimization process to quickly pinpoint the fairest models, it doesn’t force banks to choose them by design. Banks still have the final say over which models to deploy.
FairGBM
FairGBM is a constrained version of gradient-boosted trees that optimizes for both predictive performance and fairness between groups – without compromising one or the other. Because it was built on the LightGBM framework, FairGBM is fast and scalable to train models using millions of data points, an essential requirement for financial services. An open source version is also available for non-commercial and academic use to proliferate the mission of minimizing bias. Learn more about our publication here.
Whitebox Explanations
Underpinning any machine learning technique is the importance of transparent, explainable decisions. These model’s decisions need to be explainable to regulators, managers, and even consumers. All of Feedzai’s machine learning models have Whitebox Explanations – straightforward, human-readable text that justifies the model decision.
These components give banks the essential components they need to uncover biases in their models without compromising on model performance.
How Banks Benefit from Built-in Responsible AI
You can’t fix what you can’t measure. Feedzai’s built-in solutions for Responsible AI give banks the tools they need to uncover bias in their models and respond appropriately. The upside to using these tools include:
- Bias audit notebook: Quickly find and measure bias in existing models using any bias metric or attribute (e.g., age, gender, etc.).
- Fairband algorithm: Automatically discover machine learning models while incurring zero additional model training costs, all while boosting model fairness by an average rate of 93%.
- FairGBM: Improve fairness metrics by 2x without seeing a loss in detection.
- Whitebox explanations: Get transparent explanations and easily understand the driving factors behind each model’s risk decision
It’s important to note that while Feedzai helps banks identify and respond to bias in their models, banks ultimately have the final say when deploying the new models. Feedzai’s built-in Responsible AI tools give banks the choice but do not require an organization to follow its recommendations. We’ll give banks the ethical compass. It’s up to them to navigate towards their goals.
Feedzai’s assortment of built-in Responsible AI tools gives banks a roadmap to demonstrate its commitment to fairness without compromising performance. It’s a simple step in winning customer trust and long-term loyalty.
Share this article:
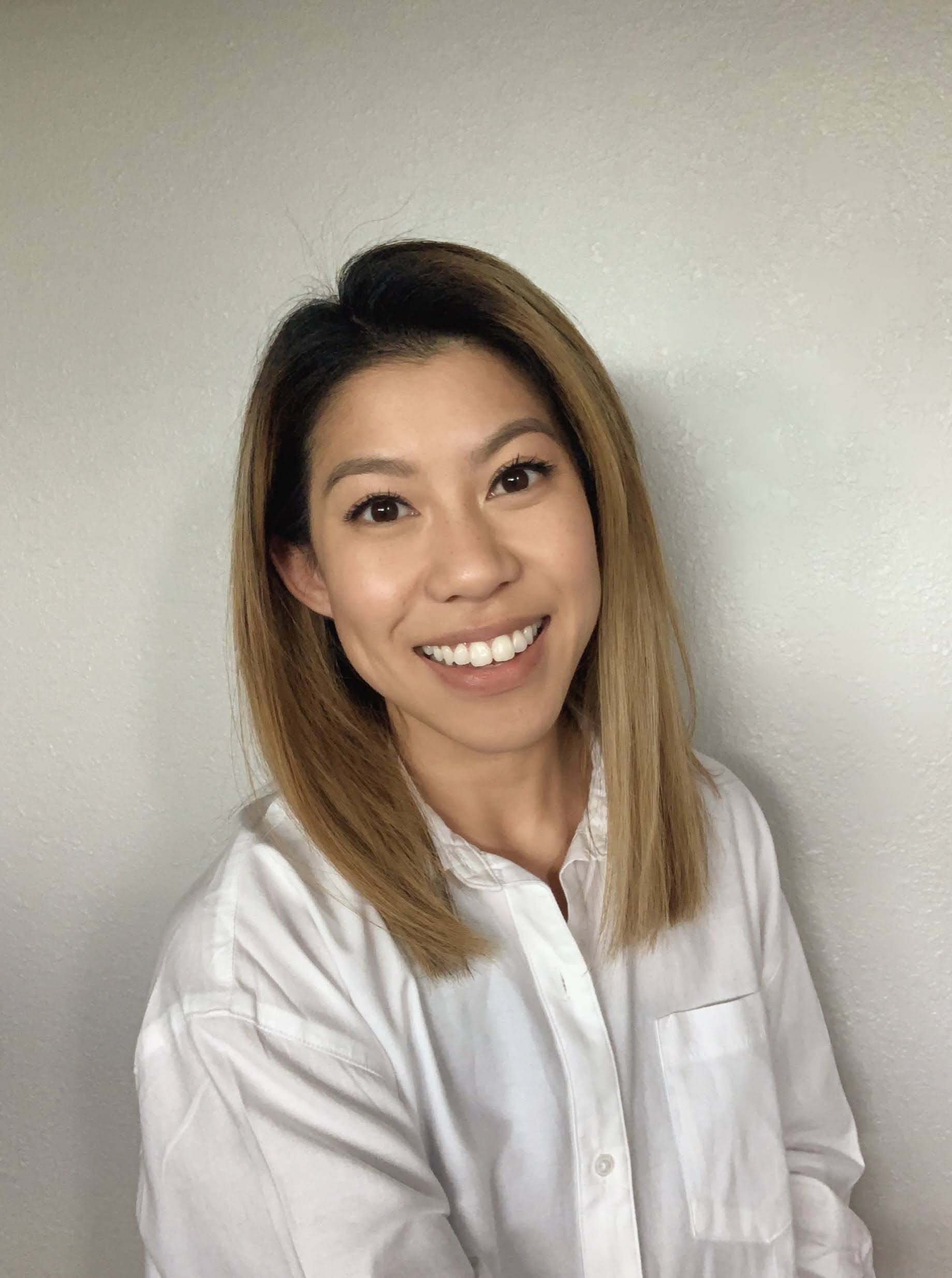
Tiffany Ha
Tiffany Ha is a Sr. Product Marketing Manager at Feedzai. She has spent the last eight years in the fraud and financial crime prevention space. Financial crime trends and tactics are always evolving, so the accompanying technology must stay ahead of the curve. She loves connecting the dots between technology and market challenges to help financial institutions achieve their goals.
Related Posts
0 Comments10 Minutes
Boost the ESG Social Pillar with Responsible AI
Tackling fraud and financial crime demands more than traditional methods; it requires the…
0 Comments8 Minutes
Enhancing AI Model Risk Governance with Feedzai
Artificial intelligence (AI) and machine learning are pivotal in helping banks and…
0 Comments14 Minutes
What Recent AI Regulation Proposals Get Right
In a groundbreaking development, 28 nations, led by the UK and joined by the US, EU, and…