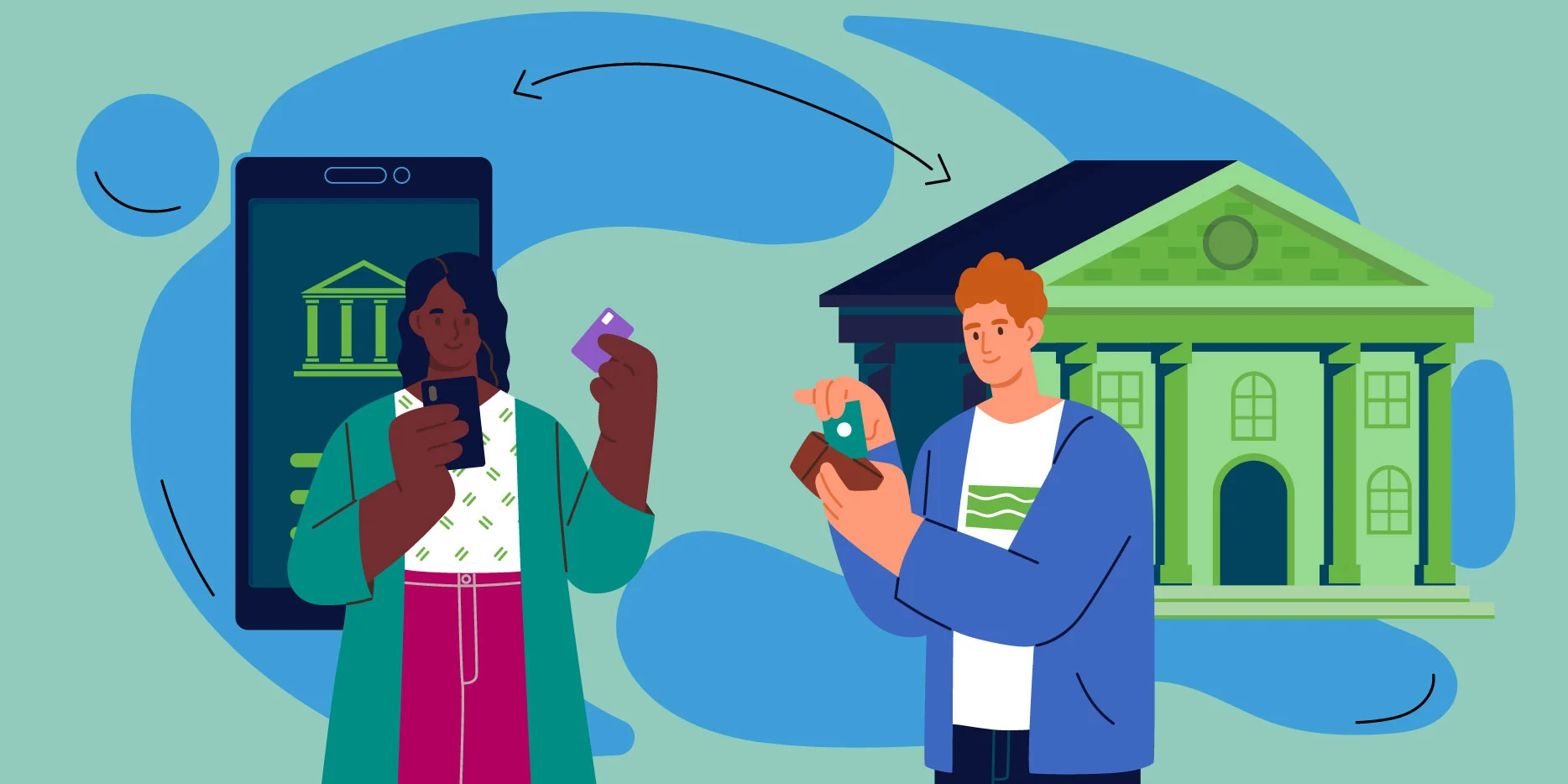
Feedzai CEO Nuno Sebastiao recently spoke with Gulru Atak Gundmen, Global Innovation Lead for Citi’s Treasury and Trade Solutions as part of the financial services firm’s Citi Talks Payments series. Among the topics discussed are how established legacy financial institutions and newer challenger banks are able to inform each other’s respective technological agendas, how Feedzai helped build the world’s first serverless bank, how artificial intelligence (AI) and machine learning can be used to build a more responsible digital society, and more.
The following is an abridged transcript of Nuno’s conversation with Citi.
At Feedzai, we manage the entire lifecycle of risk from the minute consumers onboard to get financial services, while they transact, and while they interact with the financial services systems. It’s been a journey. We started as a very small company. We’ve grown to over 500 people today covering the globe and are very, very excited with the partnerships we have.
Bridging the challenger-legacy bank gap and making banking serverless
When we were starting, we didn’t yet have the innovation movement that we have today. So essentially, large financial service organizations wanted to innovate. They wanted to think ahead and think for the consumer. Over the last three, four years, I would say we have seen newcomers, challengers some of them even sponsored by larger financial institutions (FIs), completely reinvent the way consumers interact with banking and the way consumers interact with financial services.
Today, as a company, we see ourselves supporting these two worlds. On one hand, we take the obsession with removing customer friction that is characteristic of newcomers, challengers, and FinTechs, and we take that to our more established financial services clients. Meanwhile, at the same time, we work to demonstrate to challengers and innovators the importance of regulations, compliance, and risk management at scale that we’ve learned and developed with our larger FI clients. So, everyone benefits from this ecosystem we’re working in.
In one region, we recently helped build what they call a completely serverless bank. In nine months, we helped to launch a fully regulated bank with a banking license in the local region that does not have a single server. Imagine that, or the equivalent of that, in a large FI. At the same time, we worked with them and told them they need to be absolutely clear about risk and risk management.
Why fraud is a great use case for machine learning
No matter the size, fraud is one of the most significant risks that an FI will ever face. Fortunately, fraud is also one of the more developed use cases for AI and machine learning, and for a number of reasons.
- You can make use of existing data
First, there’s a wide availability of data, historical data. We know the behavior and we know the patterns. What hasn’t happened before is making use of that data, so the data was just sitting around and not being digested properly. We realized we could apply machine learning to this, well-identified problem, starting with transaction fraud.
- You can use data to better understand behaviors
Understanding data can help you understand the customer’s journey. A good example is if you look at transaction fraud, you’re looking at one event, one payment, let’s say, and you can say this is good or bad. But if you look at the sequence of events you can say this account, or this person is orchestrating to do good or bad behavior. It’s the same data, and that data is there.
Machine learning suits itself to stopping fraud because the data is well-structured, it’s a well-identified problem and we know what we’re looking for. That’s why fraud detection and prevention is the ideal situation for the application of machine learning techniques.
- You can improve fraud prevention by sharing behaviors – not data – with other companies
Technology has evolved into a place where how we become really strong is what we learn about what we call “profiles.” Profiles are behaviors. We don’t know that a particular behavior is from Nuno or someone else. But we know that there is a behavior. With that, we manage things like GDPR or data privacy acts which are really, really important.
With those insights, when we work with the client and with the client’s data, we can say, “Okay, I’ve seen this type of profile and I know it correlates with good or bad behavior.” This is the best of both worlds. It’s not that organization A is sharing your information with organization B – I wouldn’t want that, and you wouldn’t want that. But we can share a behavior. With a profile or a fingerprint, I can identify the same profile here from a client of another institution and I’ll be able to flag it.
- You can think both globally & locally for enhanced fraud prevention
We also have the ability to ingest unique local data sets and augment them with local information that global clients have. By bringing that data into the platform, we can give you not only a global view but also a personalized one of that particular region, that particular segment of the client, and that particular institution. All of it while managing privacy concerns. That’s the power we bring to the table and that machine learning can offer.
How the pandemic has enhanced the digital society
As the world moves quickly towards becoming a digital society (accelerated by the pandemic), there’s a whole debate that can be had about this. We are all saturated with sitting at home and looking at screens like computers. But we as a society need to interact. What digitization has done is it has made things that used to be lengthy and would take forever now move faster. It’s an enabler.
But like any other technology that is an enabler, we need to ask, what we are enabling?
Are we enabling conversations? Are we enabling transactions? In financial services, we see ourselves as part of a movement that enables people who couldn’t have a banking service before because it was too expensive to serve them and banks wouldn’t have a return. Today because costs are coming down, they can get easier, affordable access to banking services.
We work with clients in regions like, for instance, in India and in other places, where just enabling that makes a difference in peoples’ lives. I believe digitization that we’re observing and has been accelerated by the current crisis do benefit many parts of society. Before some people didn’t have access to a financial service, now they do. Before they weren’t part of a global payment system, now they are.
That wasn’t possible before. When we sit down, sit back and reflect, that’s what we do. That’s the kind of change that we enable.
Machine learning has come a long way. Now the rise of mobile technology and the growth of big data are accelerating the adoption of AI and machine learning. Download our eBook Demystifying Machine Learning to understand the six stages of machine learning and how this technology can help your organization.
Share this article:
Related Posts
0 Comments6 Minutes
A Guide to Secure, Seamless User Authentication in Payments
Online payments demand a delicate balance between security and user experience. Consumers…
0 Comments7 Minutes
Combating Emerging Scams in the Philippines
The Philippines is witnessing remarkable growth in digital banking. Unfortunately, a…
0 Comments5 Minutes
Feedzai is a Leader in the 2024 IDC MarketScape for Enterprise Fraud Solutions
Exciting news! Feedzai, the world’s first RiskOps platform, is proud to have been named a…