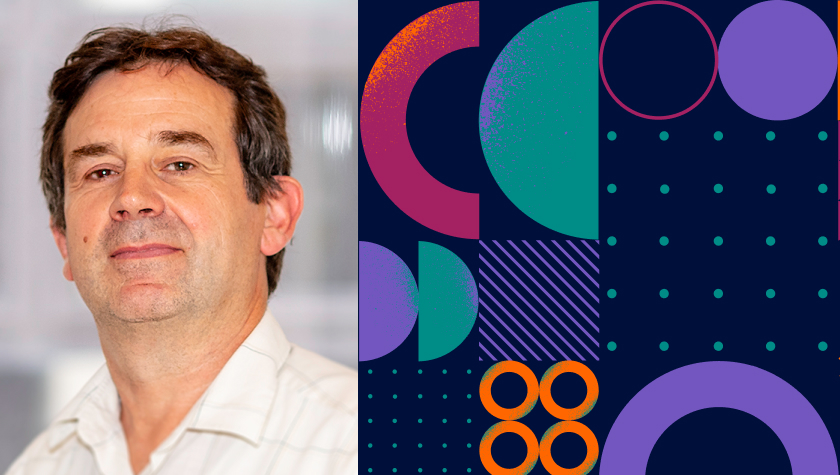
As the old saying goes, time and tide wait for no one. This is a reality the data science community understands all too well, facing an unflinching tide of new fraud threats. Fortunately, Feedzai’s Automated Machine Learning (AutoML) is turning the tide in the anti-fraud fight in data scientists’ favor and providing them more time to focus on long-term agenda items.
In the swiftly evolving realm of financial crime, data scientists cannot afford to lag. However, traditional data science workflows are laborious and time-consuming, obstructing the swift response needed to tackle new threats. Feedzai’s AutoML revolutionizes the way data scientists combat fraud, automating key elements of the data science process.
3 Key Data Scientist Challenges
Data scientists grapple with three significant hurdles in their work.
- Fraud is Always Evolving. Fraud never sits still. Bad actors are constantly innovating and testing new fraud tactics. This means just as data scientists develop machine learning models to tackle one type of fraud, newer threats have likely already surfaced.
- New Payment Channels Are Frequently Introduced. Of course, fraudsters aren’t the only ones testing new tactics. Banks and financial institutions are continually unveiling new applications and launching digital channels for onboarding. Each new application and channel requires a new set of security measures that adds to data scientists’ already heavy workload.
- Not Enough Data Scientists. As if the heavy workload wasn’t enough of a challenge, the data science community is facing a talent shortage to handle new applications across multiple channels. This causes the onboarding process to typically unfold one channel at a time.
Unpacking the Data Scientist Workflow
These challenges can delay the deployment of vital solutions by adding friction to the data science workflow. The typical workflow of a data scientist includes:
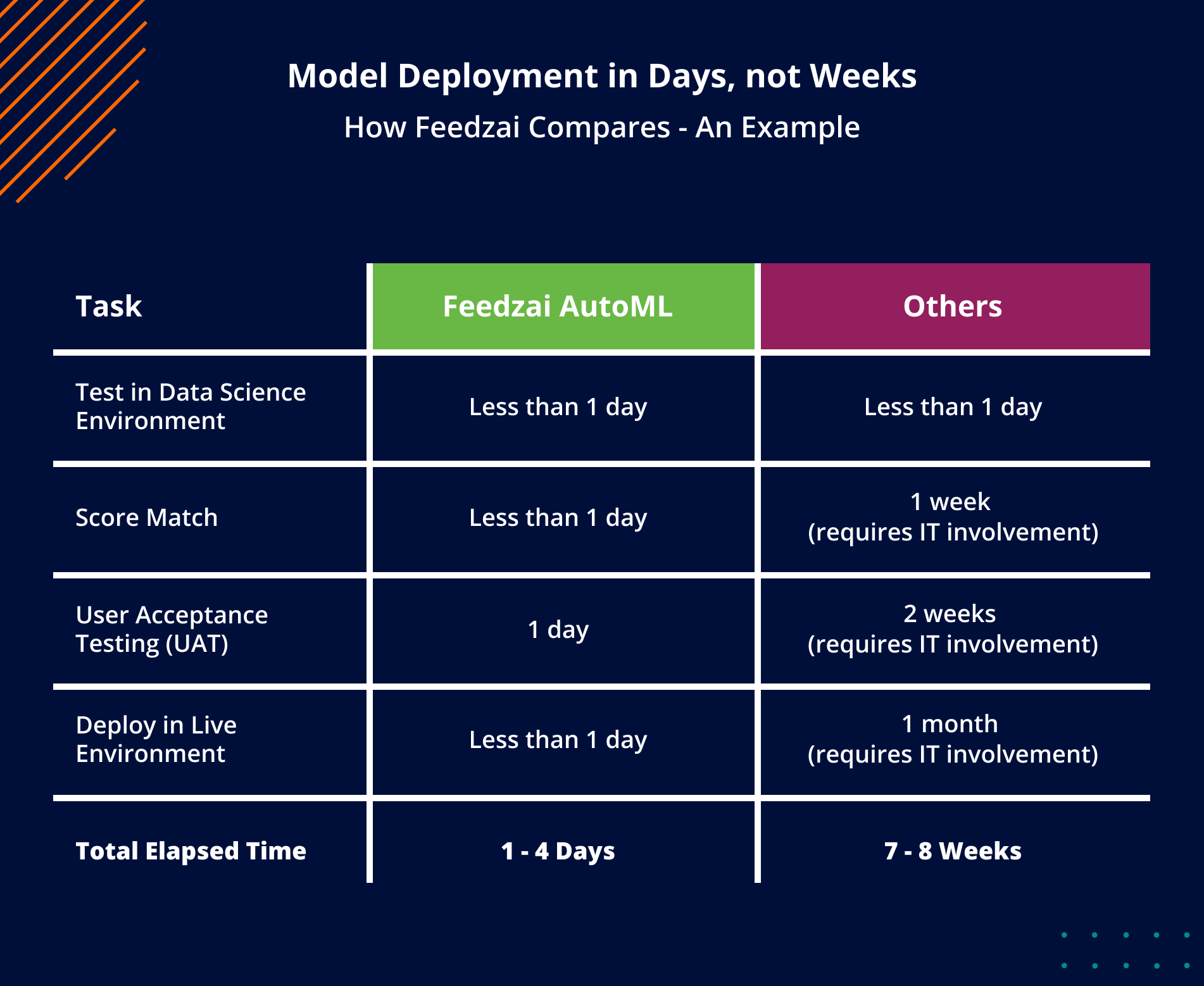
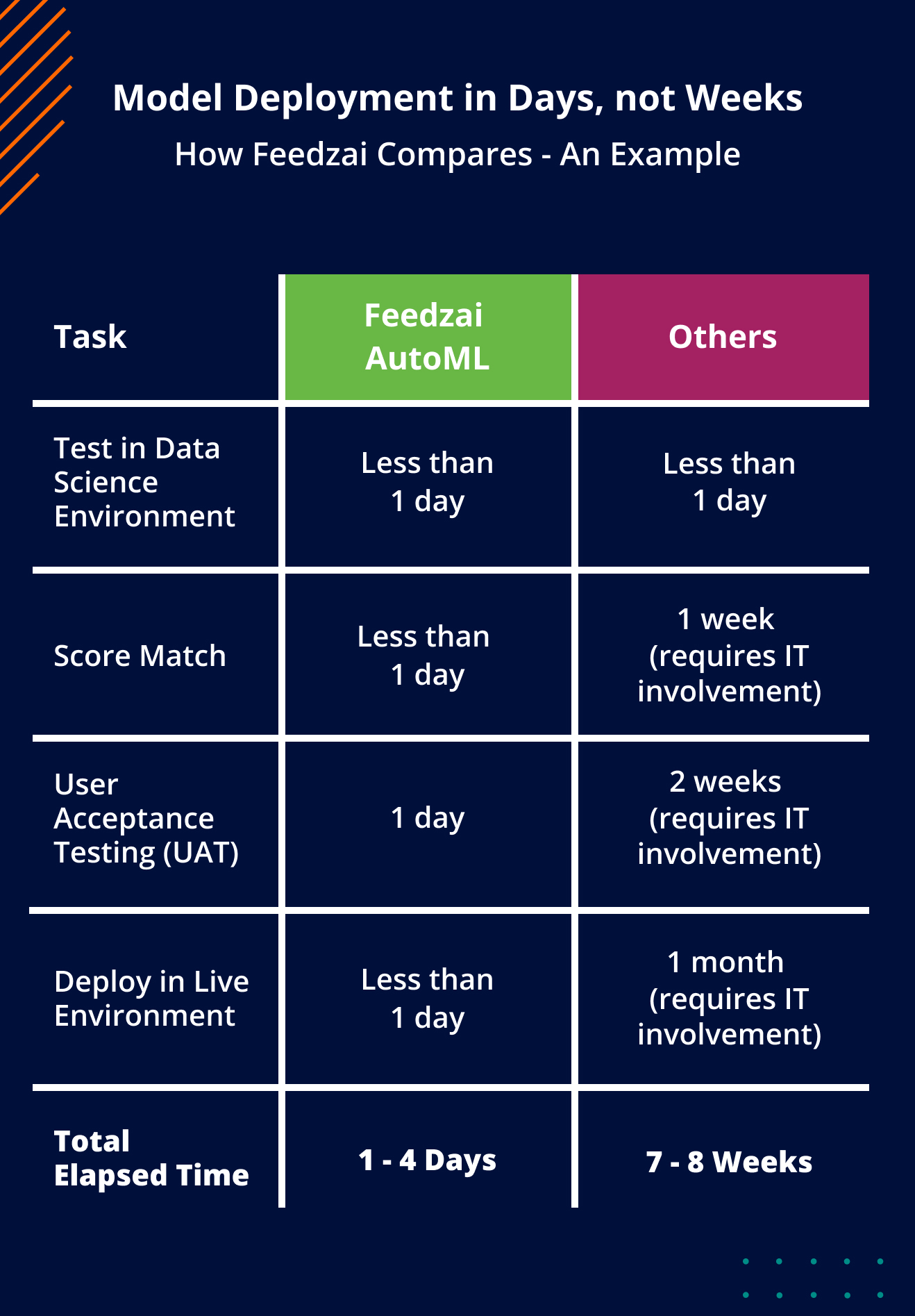
- Accessing the dataset: The required data must be obtained for model and solution building.
- Data preparation: The data is cleansed and formatted to suit the model’s requirements.
- Data exploration: The data is scrutinized to identify fraud patterns.
- Feature engineering: Features are constructed based on research to detect fraud patterns.
- Training and evaluation: Various models and configurations are trained and assessed based on the targeted performance indicators.
This multi-step workflow can extend the machine learning model deployment process and postpone the release of crucial solutions. All in all, the current process can take up to 12 weeks, require input from at least two data scientists, and distract data scientists from concentrating on essential long-term tasks.
Transforming Data Science with Feedzai’s AutoML Solution
Feedzai’s AutoML aims to address these pain points by automating critical tasks in the data science workflow, slashing deployment time from weeks to mere days. This enhancement allows data science teams to iterate models quicker and focus on more impactful tasks, like identifying new business applications and leveraging new data sources. AutoML enables a single data scientist to handle multiple use cases simultaneously, leading to faster action across several channels.
AutoML augments the data science workflow in the following ways:
Automated Data Preparation
AutoML handles data cleaning and formatting tasks, including the conversion of transactions from different countries into a preferred currency or filling in missing state fields based on zip and country codes.
Enhanced Data Engineering
AutoML uses semantic-based feature engineering, drawing on Feedzai’s extensive experience in combating financial crime, to generate meaningful domain-specific features.
Optimized Feature Selection
AutoML employs an advanced tree-based method that considers feature interactions for feature selection, mitigating overfitting and boosting model interpretability.
Automated Model Selection and Optimization
AutoML is adept at training multiple machine learning algorithms and fine-tuning models through hyperparameter optimization techniques.
OpenML Integration. Feedzai’s AutoML integrates smoothly with OpenML, offering standard APIs that allow teams to import or integrate the best open-source and third-party models into Feedzai’s platform.
AutoML provides four key enhancements to the typical data science workflow:
- Speed: AutoML expedites the data science loop, enabling quicker model iterations and deployments.
- Domain-Specific Approach: AutoML utilizes domain-specific knowledge to build effective features for the financial crime landscape.
- Extensible Solution: AutoML allows data scientists to build their features, customizing the solution further.
- Transparency: All actions and outputs of AutoML are transparent and traceable, offering data scientists complete visibility into the process.
Feedzai’s AutoML also simplifies the generation of standard model governance reports, thereby reducing report preparation time from two weeks to a few days, a boon during audits.
Feedzai’s AutoML is a game-changer in the fight against evolving fraud threats, enabling data scientists to rapidly respond to emerging fraud patterns. By automating key steps in the data science workflow, deployment time reduces dramatically, freeing up data scientists to spearhead the discovery of new business use cases and detect new fraud patterns more rapidly.
Time and tide may wait for no one. But Feedzai’s AutoML gives data scientists a much-needed tool to quickly respond to the rising tide of new fraud threats and refocus their time on long-term objectives.
Share this article:
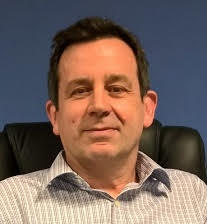
Robert Harris
Robert Harris is the Head of Product Marketing at Feedzai and a passionate proponent for fighting fraud and money laundering particularly in financial services. Robert is an accomplished leader in both small and large organizations in identifying opportunities, securing funding, and creatively delivering value in line with project goals. Whether launching new solutions or maximizing value from mature ones has a keen commercial eye and a conviction to both innovate and make prioritization decisions accordingly.
Related Posts
0 Comments6 Minutes
A Guide to Secure, Seamless User Authentication in Payments
Online payments demand a delicate balance between security and user experience. Consumers…
0 Comments7 Minutes
Combating Emerging Scams in the Philippines
The Philippines is witnessing remarkable growth in digital banking. Unfortunately, a…
0 Comments5 Minutes
Feedzai is a Leader in the 2024 IDC MarketScape for Enterprise Fraud Solutions
Exciting news! Feedzai, the world’s first RiskOps platform, is proud to have been named a…