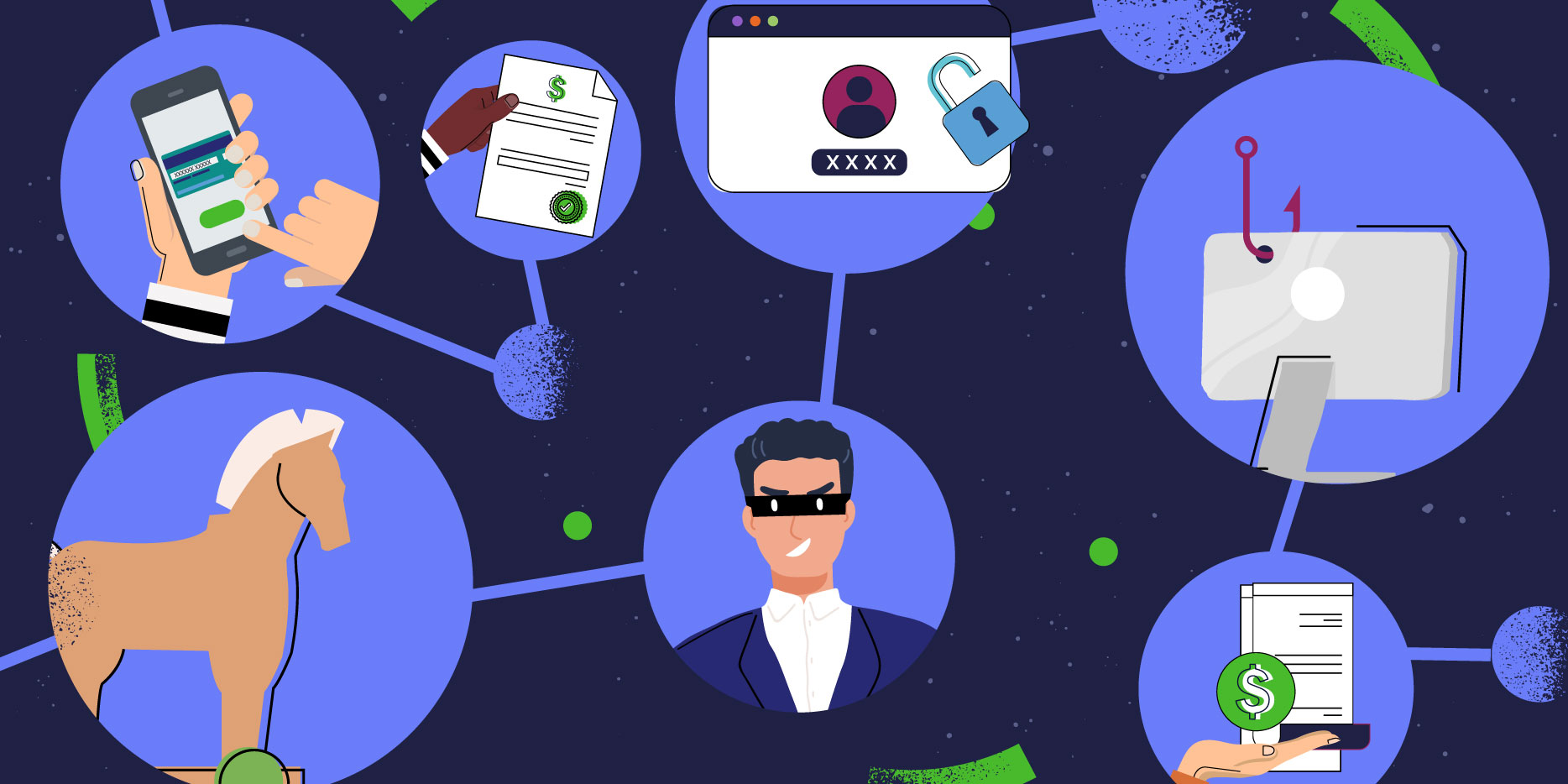
Instant transfers allow customers, as the name suggests, to move money in seconds instead of days. While this capability opens new avenues for consumers, it also comes with a very real, very risky side effect: the threat of instant fraud losses.
Once funds are delivered, fraud victims will not have time to contact their banks to stop or reverse an instant transfer. Banks, meanwhile, lack the bandwidth and resources to review numerous transactions to determine which instant transfers are fraud and which ones are legitimate. If the transfer is approved, fraudsters have immediate access to the funds, and there is little that banks or customers can do to recover the money.
The following outlines seven common types of instant transfer fraud, how they work in an instant transfer environment, and how machine learning rules can detect fraud and protect customers, employees, and businesses from falling victim to various scams.
7 types of instant transfer fraud and what to do about it
1. Authorized Push Payment Fraud
What is Authorized Push Payment Fraud?
Authorized push payment (APP) fraud occurs when a customer is tricked into transferring money to an account controlled by a criminal. This type of fraud relies heavily on the targeted individual trusting the person whom the fraudster is impersonating. The fraudster could pretend to be a representative of a victim’s bank, for example, claim that their account has been compromised and urge them to move money to a different account that they operate.
This was one of the most common types of fraud against businesses last year and is on track to continue the trend this year. APP fraud has notably risen in the U.K. market where losses reached £456 million ($603.7 million USD) last year, up from £354 million ($468.7 million USD) the previous year.
How Setting Transfer Limits Helps Stop APP fraud
The most effective way to stay vigilant against fraud is to know your customers’ normal behaviors. Understanding what constitutes normal can help your organization to realize when a customer is engaged in risky behaviors or if they are about to make a high-risk transfer. Rules-based machine learning systems can stop transfers from being completed if, for example, the number of transfers the user made in a recent span of time are far greater than the number of transfers they have made in the past few months, if the sender attempts to transfer money to several new recipients, or if the value of the transfer to new recipients is unusually high. Implementing these limits based on the knowledge of your customers’ normal behaviors can help your organization to stop fraudulent transactions from occurring.
2. Account Takeover Fraud
What is Account Takeover Fraud?
In the case of account takeover (ATO) fraud, a fraudster uses stolen credentials to access someone else’s money. Email addresses, phone numbers, or other personally identifiable information can be used to gain access to bank accounts. In other instances, fraudsters might purchase stolen login credentials from the dark web. Once fraudsters have access, they transfer money out of the breached account and into one of their own. Losses from ATO-based incidents surpassed $4 billion as recently as 2018.
Understand Your Customers’ Normal Behaviors To ID ATO Fraud.
A rules-based system can help your organization to understand the way your customers typically engage with your platform and highlight suspicious patterns. The system can then flag these patterns to identify whether an ATO event is in progress. Activities that can trigger an alert can include changes of address, frequent updates to names on an account, attempted login attempts from unrecognized mobile devices, unfamiliar geographic locations, changes in usual web browser for logins. Systems can also flag activities like money transfers to unfamiliar accounts, making purchases with new merchants, or making unusual travel arrangements.
3. CEO Fraud
What is CEO Fraud?
When your boss wants something done right away, your first instinct is to scramble in order to make it happen. Fraudsters are counting on this reaction as a means to trick employees into committing fraud on their behalf. CEO fraud works when the criminal sends an employee an email, text message, or makes a phone call impersonating the company’s CEO or another high-level executive with an urgent request.
The targeted employee is often someone who works in accounting, finance, or payroll and has the authority to approve outgoing payments. To pull off such a fraud, the criminal would first need to pull off a spear phishing or a business executive compromise (BEC) event (or both) in order to steal the executive’s credentials. These stolen credentials can be used to commit additional fraud scams, including ATO attacks.
Let the Rules Work For You
Just as they can be used to understand a customer’s normal behaviors, rules-based systems can also develop an understanding of what is normal behavior for CEOs and employees. The system’s rules can trigger alerts if an employee starts to make an unusual number of direct deposits to new recipients or if large amounts of money are being transferred faster than normal. These same rules can also determine if the user was redirected to a financial services portal from an email instead of through the desktop or mobile device they normally use. The rules can also trigger an alert if an imposter CEO email was sent to multiple employees across an organization.
4. Mandate Fraud
What is Mandate Fraud?
Mandate fraud occurs when a company employee is manipulated into redirecting a legitimate payment like direct debits or bank transfers to a fraudster-controlled account. Having acquired personal details, such as a professional contact’s name or other information, a fraudster will impersonate the representative of a supplier or an executive that the employee already knows. Next, they convince the employee to alter their payment information to a different account, possibly offering an excuse like switching FIs as a reason for the change. The scheme might not come to light until the legitimate supplier contacts the business to inquire about their missing payment. In the U.K. market, mandate fraud nearly reached £100 million ($131 million USD) in the 2018/19 fiscal year. In the U.S., the FBI reported this type of activity cost businesses $1.77 billion in losses in 2019.
Follow the Money to Detect Suspicious Behavior
Rules-based solutions can track and flag activities including when a supplier changes sensitive information like associated bank accounts. The solutions can also track the ecosystem of banks and detect suspicious patterns such as if several accounts from a single bank are making an unusually high number of payments to a single receiver account. These rules can be triggered if the number of debits from an account with no activity reported in the previous three months exceeds a set threshold.
5. Money Mule Fraud
What is Money Mule Fraud?
Money mules act as a middleman for fraudsters by making transfers on the bad actor’s behalf. There are three key types of money mule. First, an unwitting actor who has been deceived into participating in a scheme. These individuals might have been tricked by a romance or job opportunity scheme into opening up a bank account on someone else’s behalf. Second, there are witting mules who ignore the warnings of banks that they are being manipulated. These mules might remain convinced against all evidence that they stand to gain financially from the fraudster’s scheme and continue to follow instructions. Finally, there is the complicit mule who is aware of their connection to a criminal scheme. These individuals might already be profiting from fraud and travel to new markets to open accounts with legitimate banks, help criminals move money, and recruit other money mules into their scheme. The events of COVID-19 created numerous opportunities for money mule scams to target desperate individuals.
Build an Account Profile to Stop Money Mules
Organizations need to review a wide range of information in order to stay a step ahead of money mule activities. Building a profile can combine a wide range of information about a user and help organizations to detect money mule patterns before it is too late. Reviewing the profile can help organizations to build a holistic view into a user by assigning them a mule score at their application process and continuing to build on that score each time the user interfaces with the platform and different products.
In addition to building a mule score, organizations can also profile users’ behavioral data, based on information like how often they log onto a platform and if the amount they transact in fluctuates radically in a short period of time. They can also review their combined inbound and outbound payment activity, and compare monetary sources of data – including bank accounts and transaction amounts – with non-monetary sources of data, such as devices and addresses to help identify suspicious patterns that could be linked to money mule activities. Click here to find more information on how to stop money mule fraud.
6. Payroll Scheme Fraud
What is Payroll Scheme Fraud?
Payroll fraud represents roughly 8.5 percent of all global fraud, according to data from the Association of Certified Fraud Examiners. There are multiple ways for bad actors to pull off a payroll scheme and even some big-name corporations have fallen prey to this type of activity. Payroll fraud can be an inside job where an employee deliberately inflates the hours they have worked on their timesheet or submits a false overtime payment claim. In another insider scenario, a payroll employee could redirect payments from a recently terminated employee to their own account. In perhaps the spookiest example of payroll scheme fraud, companies are haunted by “ghost employees” — individuals who don’t actually work at the company but who collect a paycheck anyway. Even employers can commit payroll scheme fraud. This can happen if businesses deliberately misclassify full-time W2 employees as 1099 independent contractors in an effort to avoid payroll taxes.
Prevent Payroll Fraud by Getting to Know Your Employees
Stopping this type of fraud requires an organization to first become familiar with their employees’ normal patterns of behavior. This can be done through a two-prong approach:
In the first prong, rules-based systems can be put into place to stop known bad employee behavior, such as detecting if an employee has been sending unusually large amounts of money to new recipients, whether the number of recipients that an employee typically sends money to has increased at a much faster pace than expected, or if the sender and recipient are in completely different geographic locations.
The second prong addresses unknown employee behavior. That’s where machine learning becomes particularly useful. By building profiles around “normal” behavior, machine learning algorithms can detect deviations from baseline behavior and spot patterns that might have otherwise gone undetected.
7. Phishing
What is Phishing?
In a phishing attack, fraudsters will use a variety of tactics to deceive an individual into revealing personal information. These types of attacks could involve fraudsters using emails, phone calls, and websites and pretending to be legitimate businesses in order to deceive their target. Fraudsters often use an urgent tone of voice to convince their target to move quickly and transfer their money. These communications could contain links to fraudulent websites that have been designed to look like recognizable organizations (like eBay, for example). When the recipient clicks on the link, they can disclose their personal and payment information. This type of fraud has seen an uptick since the COVID-19 pandemic as more consumers shifted away from in-person to online commerce.
Pinpoint the Abnormalities to Protect Your Employees & Customers
Phishing attacks are similar to CEO fraud. However, instead of targeting a specific executive persona, a phishing attack can target just about anyone using email, phone calls, or SMS (a tactic known as smishing) communications. . A system that understands how these individuals behave normally on a day-to-day basis will be in a stronger position to identify if an individual or customer has been targeted by a phishing scheme. A rules-based system can detect if a sender is making more transfers than normal (or that is allowed) to new recipients or are engaging with high-risk businesses or individuals.
Key Takeaways
With payments capable of moving in a matter of seconds, it’s more important than ever to understand how fraudsters operate in order to avoid falling prey to schemes. Instant transfers are intended to serve customers and businesses, not fraudsters. That’s why it is important to learn how instant transfer fraud can be pulled off.
Rules-based machine learning solutions can help banks and businesses consider the larger picture to determine if an instant transfer poses a higher risk of fraud. The best way to prevent fraud is to ensure that you understand your customers’ normal patterns. Armed with this knowledge, machine learning rules can ensure an instant transfer is safe from fraud and secure for legitimate users.
Download the eBook Money on the Move: Understanding Traditional vs. Instant Transfers to learn more about preventing instant payments from becoming instant fraud losses. It includes handy charts detailing types of transfers across the globe.
Share this article:
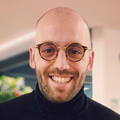
Joel Carvalhais
With over 12 years of experience in risk strategy and operations, Joel excels in defining risk prevention strategies and managing fraud on a day-to-day basis. His expertise spans banking, acquiring, eCommerce, payments, and ethical hacking. Joel is passionate about working in the risk area, driven by the thrill of identifying abnormal behaviors and catching fraudsters. Currently, he works in Product to channel his experience into delivering top-notch products with the most helpful features. His goal is to become an integral part of our customers' toolkit, providing not only insights but also essential tools.
Related Posts
0 Comments6 Minutes
A Guide to Secure, Seamless User Authentication in Payments
Online payments demand a delicate balance between security and user experience. Consumers…
0 Comments7 Minutes
Combating Emerging Scams in the Philippines
The Philippines is witnessing remarkable growth in digital banking. Unfortunately, a…
0 Comments5 Minutes
Feedzai is a Leader in the 2024 IDC MarketScape for Enterprise Fraud Solutions
Exciting news! Feedzai, the world’s first RiskOps platform, is proud to have been named a…