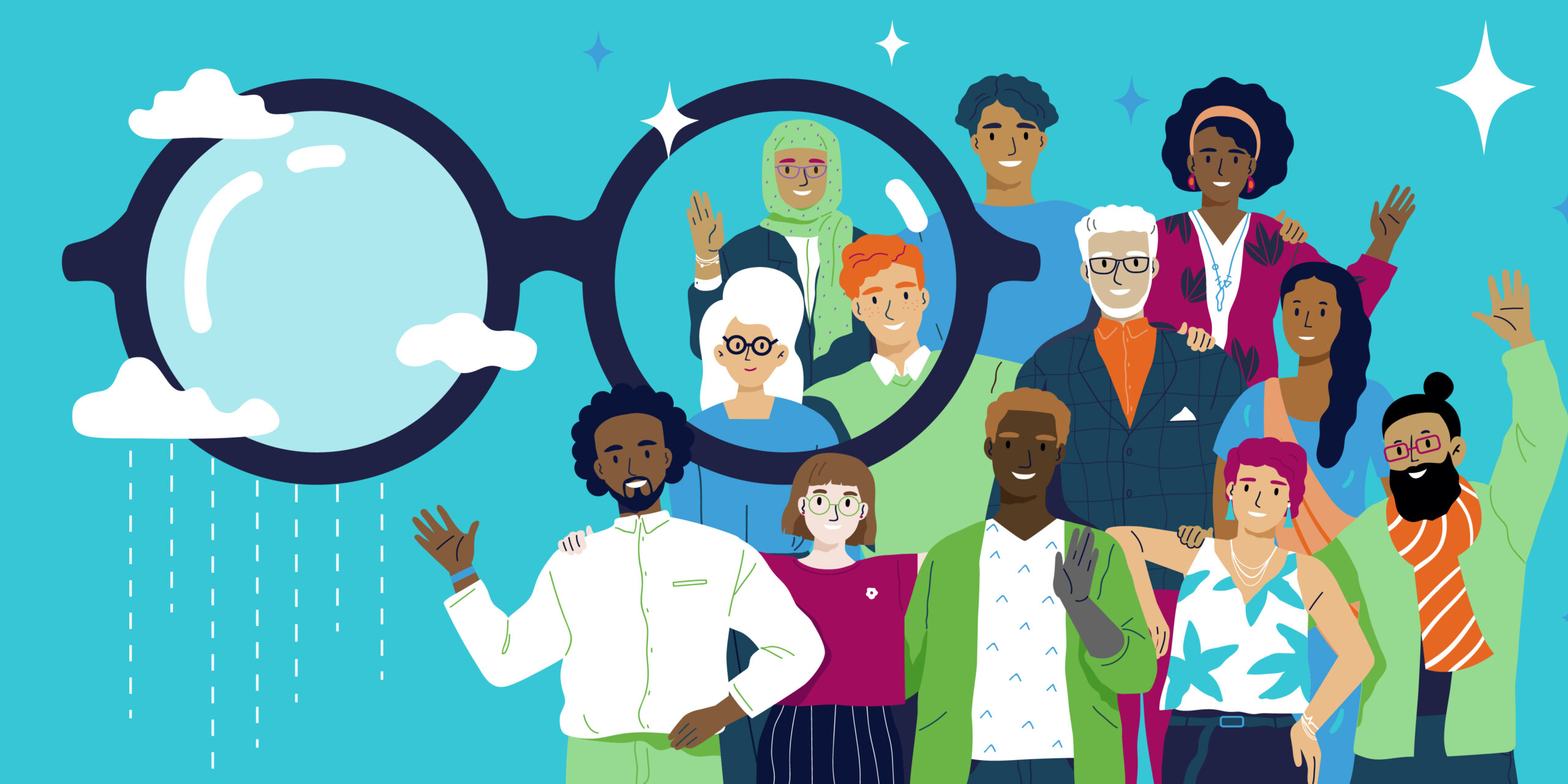
As artificial intelligence (AI) becomes increasingly used in financial services, it’s essential that financial institutions (FIs) trust the technology to work as intended and that it aligns with their ethical values. Implementing Responsible AI principles is not only the most effective way FIs can protect their customers and their brand from misbehaving AI - it’s also the right thing to do.
Why Responsible AI Matters
It is very easy, even unintentionally, to develop AI that does not work in production as you expect. The model that performed really well on the test dataset can make discriminatory decisions when in production, disproportionately hurting end customers from certain groups. Or if the model is not robust enough to respond to changes in the data – like a new bot attack – you will see an unexpected spike in fraud losses.
FIs count on AI and machine learning to enhance decision-making for a wide range of use cases, from customer relationship management to lending to new bank account applications or payments fraud detection. However, no organization should blindly rely on black-box AI.
AI bias often only becomes clearer by checking its impact on smaller groups. An FI’s false positive rate for New York City might look good, for example. But when looking more closely at where customers live, the FI might discover their algorithm declines considerably more legit credit card transactions from Brooklyn residents than Manhattan residents – regardless of whether fraud really occurs more often in one area than the other. In the effort to prevent fraud, banks have allowed bias to infiltrate their system, leaving legitimate customers vulnerable to discrimination.
What is Responsible AI?
Responsible AI is a strategic approach to develop and run AI-based applications that empower organizations to manage their ethical risks. Responsible AI follows ethical principles – including fairness, privacy, transparency, reliability, accountability – serving as guardrails to AI risks. These principles are intended to ensure AI reaches fair, inclusive decisions for all customers, offers understandable explanations for how its decisions are reached (as opposed to a black box solution), and holds teams accountable for the system’s behavior. The system should also be kept secure, make privacy a priority, and demonstrate reliability and safety.
These principles should be embedded not only in technical processes but also in people processes. When creating a new AI-powered application, FIs should consider how it affects people and assesses ethical risks at each step of the project, from scoping to maintenance.
It’s important to note that Responsible AI isn’t a silver bullet that washes away bias forever. As these systems run continuously, bias can creep in at any time. In this respect, bias is like cholesterol: it is easy to take in without realizing it – and hard to remove. That’s why Responsible AI requires continuous attention and informed decision-making.
Common Misconceptions
Of course, doing the right thing is often harder than it sounds. Although AI is now a commodity in several industries, including financial services, most organizations are inexperienced at managing its risks. Too often the work, expertise, and resources it takes to make Responsible AI a reality seems too overwhelming. Some FIs fear they will have to refactor their machine learning pipeline to address ethical AI issues. And it’s often unclear how effective ethical AI models will be at preventing and detecting fraud.
Fortunately, Responsible AI doesn’t have to be an either/or decision. It’s time to understand that Responsible AI is both attainable and efficient for FIs.
Many executives are under the false impression that focusing on responsible AI is too expensive, undermines the FI’s fraud detection capabilities, or that the problem lies entirely in the data. Let’s dispel these common misconceptions about Responsible AI.
Misconception 1: It’s Expensive to Focus on Bias and Fairness
Misconception: Addressing AI Fairness in machine learning models is a costly endeavor that ultimately results in more fraud and greater fraud losses.
Reality: By defining fairness requirements and objectives, we can assess biases in the datasets, collect more data (if needed), and apply bias reduction techniques to train fairer models without sacrificing much predictive power or incurring in additional training costs.
Misconception 2: Focusing on Responsible AI Greatly Compromises Model Performance
Misconception: Adjusting machine learning models to treat all groups fairly will result in a significant reduction in performance (e.g., lower fraud detection).
Reality: It’s possible to improve the fairness of your machine learning models by sacrificing a small fraction of fraud detection accuracy. Improving the robustness and explainability will result in better performance when the model is in production.
Misconception 3: Bias is in the Data, Not the ML Pipeline
Misconception: Bias comes from upstream when data is collected or sampled.
Reality: While biases can be introduced in the data, they can also be introduced in the ML pipeline. Even a non-biased dataset can generate biased decisions. That’s why model practitioners can’t simply place responsibility for AI bias upstream to the data collectors.
Now is the Time to Focus on Responsible AI
Here are a few reasons banks should be focusing on making AI fairness a priority, beyond costs and operational considerations.
Reason 1. It’s the Right Thing to Do
Responsible AI is more than just a buzzword or a fad that the financial services sector can weather. It’s the cornerstone of the industry-wide mission to ensure that FIs consistently make fairer, ethical decisions that ultimately make a positive impact on peoples’ lives. FIs that commit to a fairer AI framework can rest assured that when automated decisions are made, they are much less likely to unfairly deny loans or stop people from paying their bills because of their race, gender, age, or where they live.
Reason 2. Customers Respect Socially-Conscious Brands
Positioning your organization as proudly socially responsible is a strong value proposition in courting Millennials and Gen Z consumers. Both groups take social responsibility very seriously. Recent research found 83% of Millennials are loyal to companies that contribute to social issues they care about. Another survey found 70% of Gen Z try to do business with companies they consider ethical. FIs that take the lead on demonstrating their Responsible AI commitment have an opportunity to distinguish themselves from their competitors.
Reason 3: Start Now, Don’t wait for Regulators
Think of how safety innovations began appearing in cars and trucks. When concepts like seat belts and airbags first debuted they were seen as intrusive. Fast forward to today and it’s hard to imagine a consumer who would willingly drive a car without these features. Automakers that had already added rearview cameras, automatic braking, and blind-spot detection to their vehicles were ahead of the curve. This makes it easier to win over safety-oriented consumers. Having won over consumers with their innovations, regulatory agencies eventually began requiring automakers to build their new models. Just as car manufacturers who had already invested in these technologies were in a much better position than their competitors, FIs that implement Responsible AI now will be in a stronger position when AI becomes regulated by government agencies.
FIs that use AI that interacts directly or indirectly with people should assess the risks of each application and implement operational controls and mitigation strategies. Regardless of existent or future regulations, without basic controls, FIs are running the risk of using misbehaving AI that can hurt people, amplify societal biases, and create discriminatory obstacles to accessing financial services (even if indirectly through blocking transactions based on a model’s fraud score).
Reason 4: You’ll Protect Your Reputation
Reasons 1 through 3 on this list are carrots, but reason 4 is a stick. If you’re hesitant to invest in Responsible AI because you believe it’s too complicated, consider the alternative. Your FI will face a considerable public backlash if it’s discovered that your current AI and machine learning models have been discriminating against certain groups. The fallout could include lawsuits from affected parties, fines and audits from regulators, and a badly battered public image.
The bottom line is, FIs have a responsibility to address the biases that may have infiltrated their machine learning models. Responsible AI is not only the right thing to do. It’s a goal that is within reach. Let’s work together to do the right thing and make Responsible AI a priority.
Does your AI reflect your organization’s ethical values? Does it offer an inclusive experience for all of your customers? If you’ve got questions about Responsible AI, we’ve got answers. On May 26, join this live cutting-edge webinar Responsible AI in Financial Crime Prevention with Feedzai’s Pedro Bizarro, Pedro Saleiro, and Andy Renshaw to learn more about the real-world scenarios for Responsible AI, how to mitigate AI bias, and more.
Share this article:
Related Posts
0 Comments15 Minutes
ChatGPT Revolutionizes Retail Banking…Soon
The world is buzzing with excitement about Large Language Models (LLM) and Generative AI…
0 Comments11 Minutes
ChatGPT Creates New Data Science Challenges
ChatGPT can spit out a fraudulent email from Amazon in a matter of seconds. It can pass…
0 Comments8 Minutes
Understanding FairGBM: Feedzai’s Experts Discuss the Breakthrough
Feedzai recently announced that we are making our groundbreaking FairGBM algorithm…