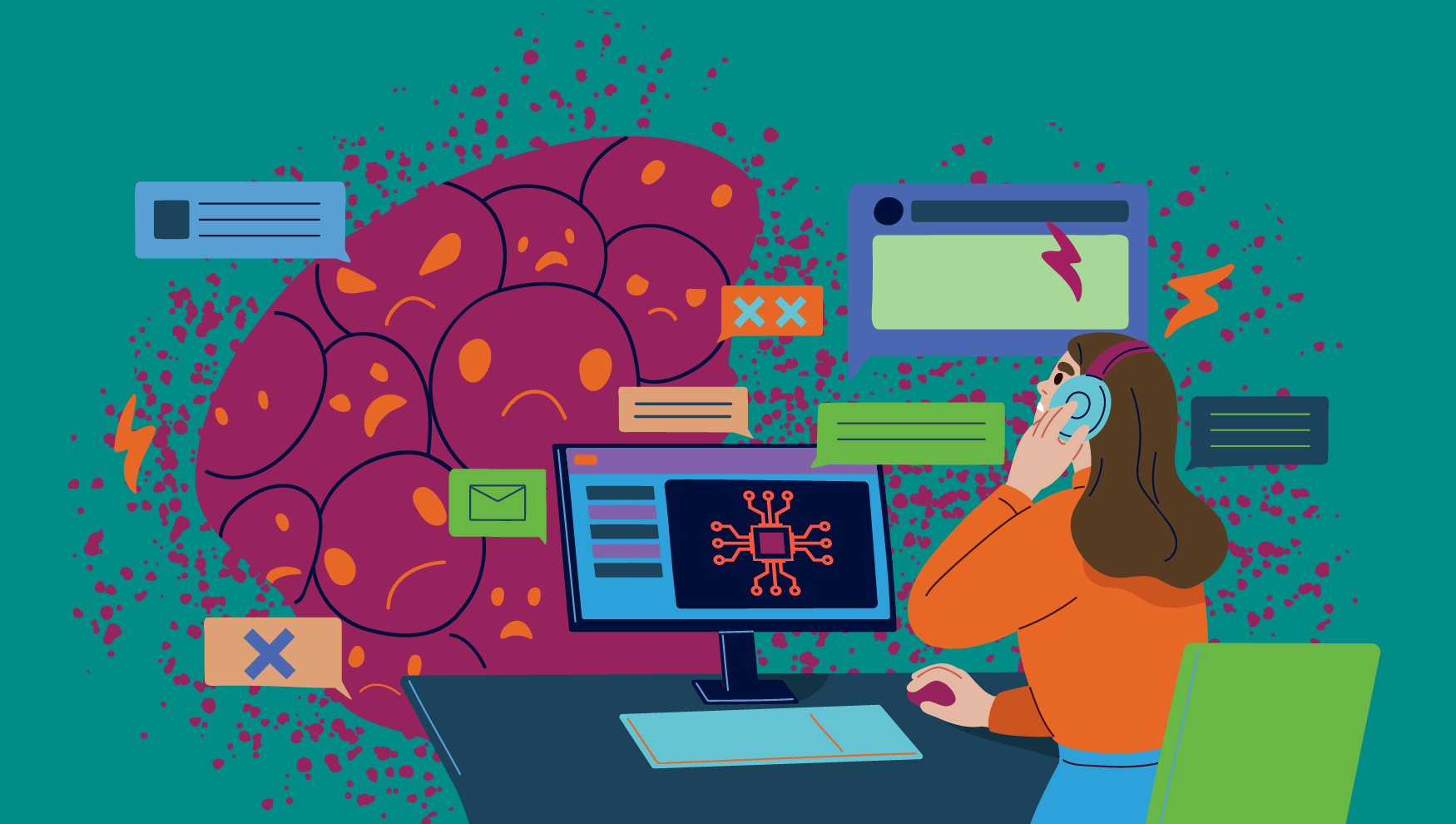
The world is buzzing with excitement about Large Language Models (LLM) and Generative AI thanks to ChatGPT – the new cool (and scary, for some) AI technology!
ChatGPT generates human-like text based on prompts it receives, and can even write poems. Dozens of articles have been published speculating on how Generative AI will change the world. From replacing Google to the advent of artificial general intelligence (AGI), there’s no shortage of predictions.
As a bank, you may rightly wonder if you can implement ChatGPT in particular and generative AI, in general, to improve your customer experiences and increase operational efficiencies.
The good news is that you can likely do both of these things – and here’s the important part – in time. If an ounce of prevention is with a pound of cure, then now is a critical time to take a step back and understand the current limitations and risks of ChatGPT. Once you know that, you will know what is needed to be built on top to use it safely in retail banking.
Understanding ChatGPT’s Risks, Limitations, and Usefulness for Retail Banking
What better place to understand ChatGPT than to “speak” to it myself? I interviewed ChatGPT about its potential role in retail banking. My questions focused on Responsible AI, a set of principles and practices to develop AI innovations responsibly, and also an important tool in a bank’s Environmental, Social, and Governance (ESG) initiatives.
Below are the prompts I gave ChatGPT, and its answers. Additionally, I’ve provided a brief analysis of its answers to each question to illustrate the strengths, weaknesses, and potential risks of generative AI.
Explain Generative AI, Large Language Models, and ChatGPT
Generative AI refers to a type of artificial intelligence that is capable of generating new and original content, such as images, text, music, and more. This is in contrast to other forms of AI that are designed to recognize, classify, or process existing data.
Large Language Models (LLMs) are a type of generative AI that use deep learning algorithms to analyze and generate text. They are trained on massive amounts of text data and can be used for a variety of tasks, such as language translation, question answering, and text generation.
ChatGPT is a specific type of large language model developed by OpenAI. It is designed to respond to user input in natural language, making it useful for applications such as customer service chatbots, virtual assistants, and text-based games.
Grade: A. ChatGPT provided a straightforward answer for a general audience, and didn’t get too technical. Moreover, the answer was both accurate and truthful.
How can banks use ChatGPT in retail banking?
ChatGPT can be used in retail banking in several ways:
Customer Service: ChatGPT can be integrated with banking websites or mobile apps to provide 24/7 customer support. Customers can get quick answers to their queries without having to wait for a human representative.
Fraud Detection: ChatGPT can be used to analyze customer transactions and detect suspicious activity, flagging potential fraud in real time.
Personalized Marketing: ChatGPT can be used to generate personalized product recommendations and marketing messages based on customer data and behavior.
Investment Advice: ChatGPT can be integrated with robo-advisory platforms to provide personalized investment advice to customers based on their financial goals and risk tolerance.
Loan Processing: ChatGPT can be used to automate the loan processing and approval process, reducing the time and effort required to process loan applications.
Chatbots: ChatGPT can be used to build conversational chatbots that can handle customer queries and provide relevant information, improving the overall customer experience.
Again, ChatGPT provides an answer that appears confident. However, it lacks depth. A user must be an expert and provide nuanced prompts to gain a more complete picture. To accomplish this, I changed the prompt to focus on safety.
Is it safe to use ChatGPT in retail banking? What are its limitations and ethical risks?
No, it is not safe to use ChatGPT directly in retail banking. ChatGPT is an AI language model that is not designed to handle sensitive financial or personal information and may not have the necessary security measures to protect this data.
The limitations and ethical risks of using ChatGPT in retail banking include:
Security: The lack of built-in security measures to protect sensitive financial and personal information.
Accuracy: ChatGPT may provide incorrect or incomplete information, which could result in financial harm to customers or banks.
Bias: ChatGPT is trained on a large dataset and may reflect societal biases in its responses, leading to discriminatory outcomes in retail banking.
Responsibility: The use of ChatGPT in retail banking raises questions about accountability and responsibility for its actions and decisions, particularly in the event of harm to customers or financial losses.
Regulation: There may be legal or regulatory restrictions on the use of AI technology in retail banking.
Given these limitations and ethical risks, it is recommended to use ChatGPT technology as part of a larger system with appropriate security measures and to be transparent with customers about the limitations and potential risks of using AI in retail banking.
ChatGPT seems to have generic safeguards and it answers cautiously about its safety. Let’s dig a little deeper to gain an adequate understanding of the risks involved.
What type of bias might ChatGPT have, and how can it harm retail banking customers?
ChatGPT, being an AI language model trained on a large dataset, may reflect various types of biases that exist in the data it was trained on. These biases can harm retail banking customers in different ways:
Demographic bias: If the training data reflects a biased distribution of demographic characteristics, ChatGPT may produce discriminatory outcomes in retail banking, such as denying loans or other financial products to certain groups of people based on their race, gender, or other demographic factors.
Conceptual bias: If the training data reflects biased assumptions about what constitutes a typical customer or typical financial product, ChatGPT may make incorrect assumptions about customers’ needs and preferences, leading to poor recommendations or decisions.
Historical bias: If the training data reflects historical patterns of discrimination or bias in financial services, ChatGPT may produce discriminatory outcomes in retail banking, such as denying loans or financial products to certain groups of people.
Algorithmic bias: If the algorithms used to train ChatGPT reflect biased assumptions or techniques, it may produce biased outputs in retail banking, such as making discriminatory decisions or predictions.
These biases can result in harm to retail banking customers, such as denial of loans, overcharges, and other financial losses, as well as violations of privacy and discrimination. It is important to address these biases and to take steps to ensure that AI systems used in retail banking are fair, transparent, and accountable.
It’s an excellent response that summarizes the harm caused not just by large language models trained on potentially biased data, but on all machine learning algorithms that do not employ a responsible AI approach.
How can Responsible AI make Generative AI safer for future use in retail banking?
Responsible AI practices can help make Generative AI, including language models like ChatGPT, safer for use in retail banking and other industries by reducing the risks associated with their deployment. Some ways that Responsible AI practices can help include:
Aligning AI with human values and ethical principles: Ensuring that the AI systems are designed and trained to align with human values, ethical principles, and the interests of stakeholders, can help mitigate potential harm.
Monitoring and mitigating bias: AI systems can contain biases, which can harm certain groups of people or perpetuate harmful stereotypes. Responsible AI practices can help identify and mitigate these biases through regular audits and evaluations of the training data, models, and outputs.
Ensuring transparency and interpretability: Making AI systems transparent and interpretable can help users understand how the AI is making decisions, which can increase trust and reduce the risks associated with their deployment.
Providing human oversight and control: Including human oversight and control mechanisms in AI systems can help mitigate the risks of unintended consequences, and allow for intervention if necessary.
Adopting responsible data management practices: Responsible data management practices, such as protecting privacy and ensuring data security, can help mitigate the risks associated with the deployment of AI in retail banking and other industries.
By adopting Responsible AI practices, AI developers and organizations can help make Generative AI safer for future use in retail banking and other industries, and increase the benefits and minimize the risks associated with their deployment.
The important takeaway here is that I knew to ask about Responsible AI. Many think that ChatGPT will provide them with all the answers. But it can’t answer questions people don’t think to ask. Human expertise and partnership are essential in extracting the most benefits from any technology.
Can I trust ChatGPT’s answers?
ChatGPT is an advanced language model developed by OpenAI that generates text based on patterns it learned from a vast corpus of text data. However, the accuracy and reliability of its answers depend on several factors, such as the quality of the training data and the context of the input.
While ChatGPT has been trained on diverse text data, it may still contain biases and inaccuracies inherent in the training data. Additionally, its responses may not always be accurate, complete, or up-to-date.
It’s essential to evaluate the responses generated by ChatGPT critically and not blindly trust the information it provides.
It’s recommended to use ChatGPT as a tool to generate text or information, but not as a sole source of truth or advice.
It’s always a good practice to verify the information generated by ChatGPT with other sources and consult with experts where necessary.
A notable issue with ChatGPT is that it does not cite its sources. Imagine spouting information as fact without being able to produce evidence of that fact. It might work in politics, but not in retail banking.
Generative AI and Large Language Models Like ChatGPT Will Revolutionize Retail Banking
Generative AI will change the world. It’s a bold statement, but arguably anyone reading the above “conversation” will agree. You know what else we all pretty much agree with? Change is scary. It can also be absolutely incredible. It can benefit humans for generations to come.
I embrace the change Generative AI, powered by foundational models like large language models, will bring wholeheartedly and cautiously. At this stage, the risks of deploying foundational models without tailored safeguards to retail banking customers still outweigh the benefits. Some of the limitations and risks include untruthful content, privacy leaks, biases, and lack of transparency. But that will improve.
If there is one great takeaway from investigating the rapid development of large language models it is that right now – right this very second – banks must invest in Responsible AI. Implementing Responsible AI tooling and methodologies will enable the safe adoption of innovations in generative AI that will improve customer experiences, improve operational efficiencies, and fulfill ESG obligations.
Share this article:
Related Posts
0 Comments11 Minutes
ChatGPT Creates New Data Science Challenges
ChatGPT can spit out a fraudulent email from Amazon in a matter of seconds. It can pass…
0 Comments8 Minutes
Understanding FairGBM: Feedzai’s Experts Discuss the Breakthrough
Feedzai recently announced that we are making our groundbreaking FairGBM algorithm…
0 Comments5 Minutes
Feedzai Releases and Open Sources FairGBM Algorithm
Today, we’re thrilled to announce the next innovation in our commitment to delivering…