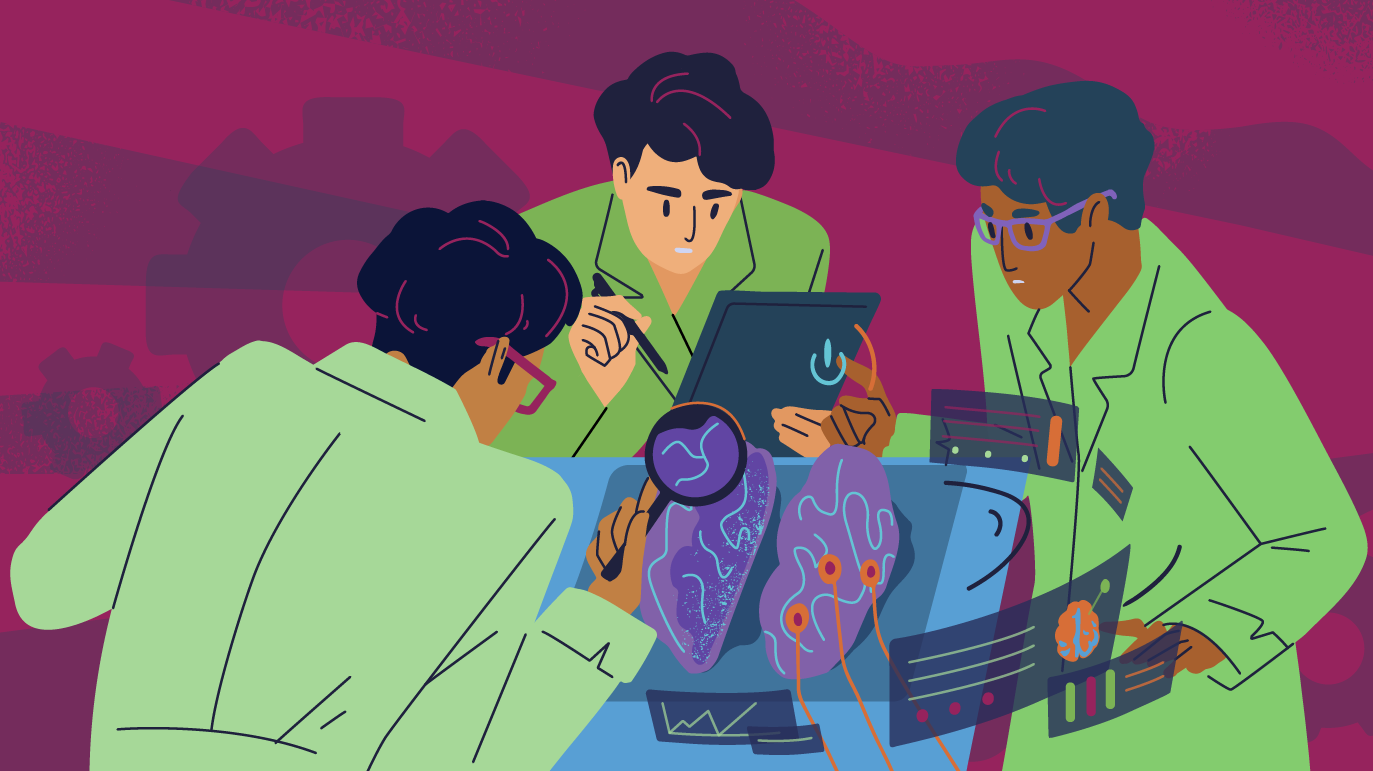
It’s always better to learn about a problem before it spirals out of control. When your car has trouble, a “check engine” light flashes prompting you to visit a mechanic. If there’s smoke in your home, a smoke alarm alerts you of the danger. For financial institutions (FIs), Feedzai’s Feature Investigation alerts Data Science teams of issues with the organization’s data before they become too big to address.
Feedzai’s Feature Investigation Automatically Detects Data Drift
By now, you’re probably familiar with the “how it started vs. how it’s going” social media trend. If not, here’s a quick summary. Social media users share a photo of themselves in the early stages of a relationship. Next, they’ll post a more recent one to demonstrate how things have changed since the relationship began.
Financial data patterns constantly experience their own “how it started vs. how it’s going” comparisons. Models are trained with historical transactional data, which is assumed to represent the data an FI will encounter in future transactions. But data patterns change over time for various reasons, a phenomenon known as data drift.
FIs Face a Data Drift Challenge
Some changes stem from consumers rapidly changing their financial habits, as seen during the pandemic. Other changes are the result of fraudsters exploring new approaches to commit fraud. As these changes unfold, the data used to train the models may no longer be representative of future transactions, impacting the performance of these models.
For example, let’s say a bank has a model in production that identifies risky transitions. But in the past week, the number of alerts is unusually higher than expected. This is often due to one or more of the model’s data features behaving differently in production compared to the historical data used to train the model. Discovering the cause of the discrepancy is difficult at best. The data often contains hundreds or even thousands of features. Narrowing down the features behind the data drift can be a challenging and time-consuming task for data scientists.
Feedzai’s Feature Investigation solves this problem by automatically analyzing the behavior of each data feature over time and alerting team members when there is a concerning drift. The system, built on our AI Observability framework, provides visibility on how models and features perform in production and empowers FIs to quickly fix issues before they balloon out of control.
Interactively Visualize Data Drifts
Data visualization is a critical element of Feedzai’s feature investigation. The system’s visualization capabilities are split into three parts.
- Features Overview. The system presents an overview display with a heatmap outlining which features have changed the most in comparison with the training period. Using this view, data scientists can seamlessly narrow down the features which need further investigation.
- Single Feature Review. Feedzai’s feature investigation also allows teams to focus on specific features individually. Once data scientists have used the heatmap to determine which features require further investigation, they can look at those features more closely to understand how they have changed over time. This investigation can also reveal upstream and downstream feature dependencies, allowing the data scientist to have a more complete picture of the data drifts.
- Feature Histogram. Finally, Feedzai’s feature investigation includes a feature histogram – a visual representation of how data has shifted over time versus a reference period, for a specific field.
A Roadmap for Proactive Feature Investigation
Feedzai’s feature investigation improves our clients’ risk strategy by preventing their services from falling victim to fraudulent attacks while delivering top-notch customer service. By collaborating with our team, FIs gain both a valuable tool and resources that enable their own data science teams to automatically detect data drift and quickly respond to it.
Real-World Feature Investigation Results for a Large European Bank
Feedzai’s feature investigation has positively impacted the risk strategy of one of our clients, a large European bank. After deploying feature investigation, the bank was able to uncover several major issues with its data that had previously gone undiscovered. This included missing data values for certain features and different value distributions for other features that indicated new consumer behavior not present during the reference period.
It only took the feature investigation system one day to bring these issues to light which resulted in immediate actions: fix the data ingestion issues from the bank’s side and retrain the model with the most recent data.
It’s always better to learn about a problem before it balloons into something bigger. With the availability of Feedzai’s ‘feature investigation, our clients have an automated tool that enables them to quickly respond to data drift patterns. Proactively responding to broken features and new patterns is critical to stay ahead of shifting consumer – and fraudster – behavior.
Are you concerned about data drift and responding to new fraud patterns? Schedule a demo with us today to see how our experts and our technology can help establish digital trust for you and your customers.
Share this article:
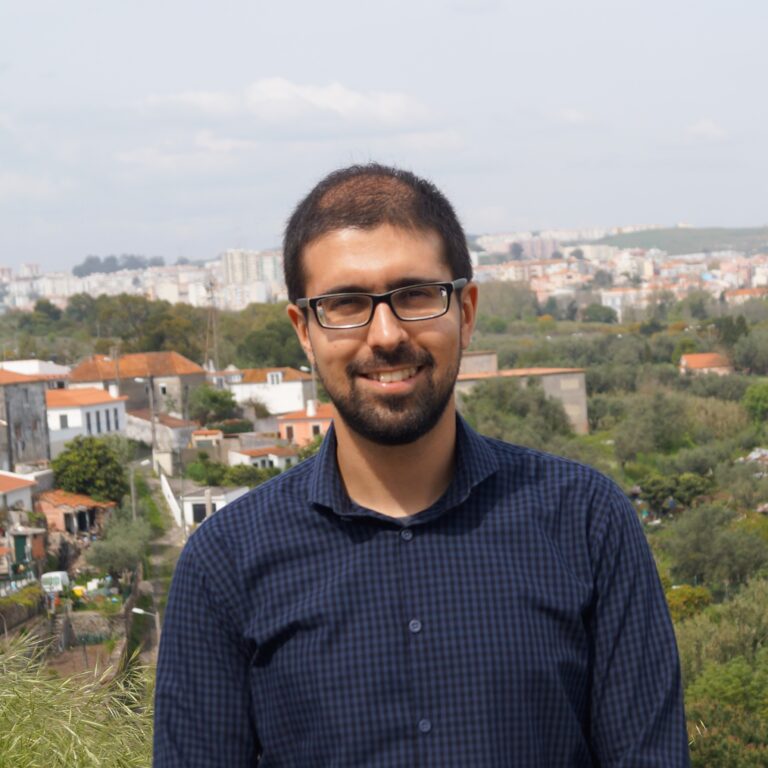
Hugo Ferreira
Hugo Ferreira is a Senior Manager of AI Research at Feedzai. He loves to develop state-of-the-art AI to build innovative solutions for financial risk prevention.
Related Posts
0 Comments10 Minutes
Boost the ESG Social Pillar with Responsible AI
Tackling fraud and financial crime demands more than traditional methods; it requires the…
0 Comments8 Minutes
Enhancing AI Model Risk Governance with Feedzai
Artificial intelligence (AI) and machine learning are pivotal in helping banks and…
0 Comments14 Minutes
What Recent AI Regulation Proposals Get Right
In a groundbreaking development, 28 nations, led by the UK and joined by the US, EU, and…