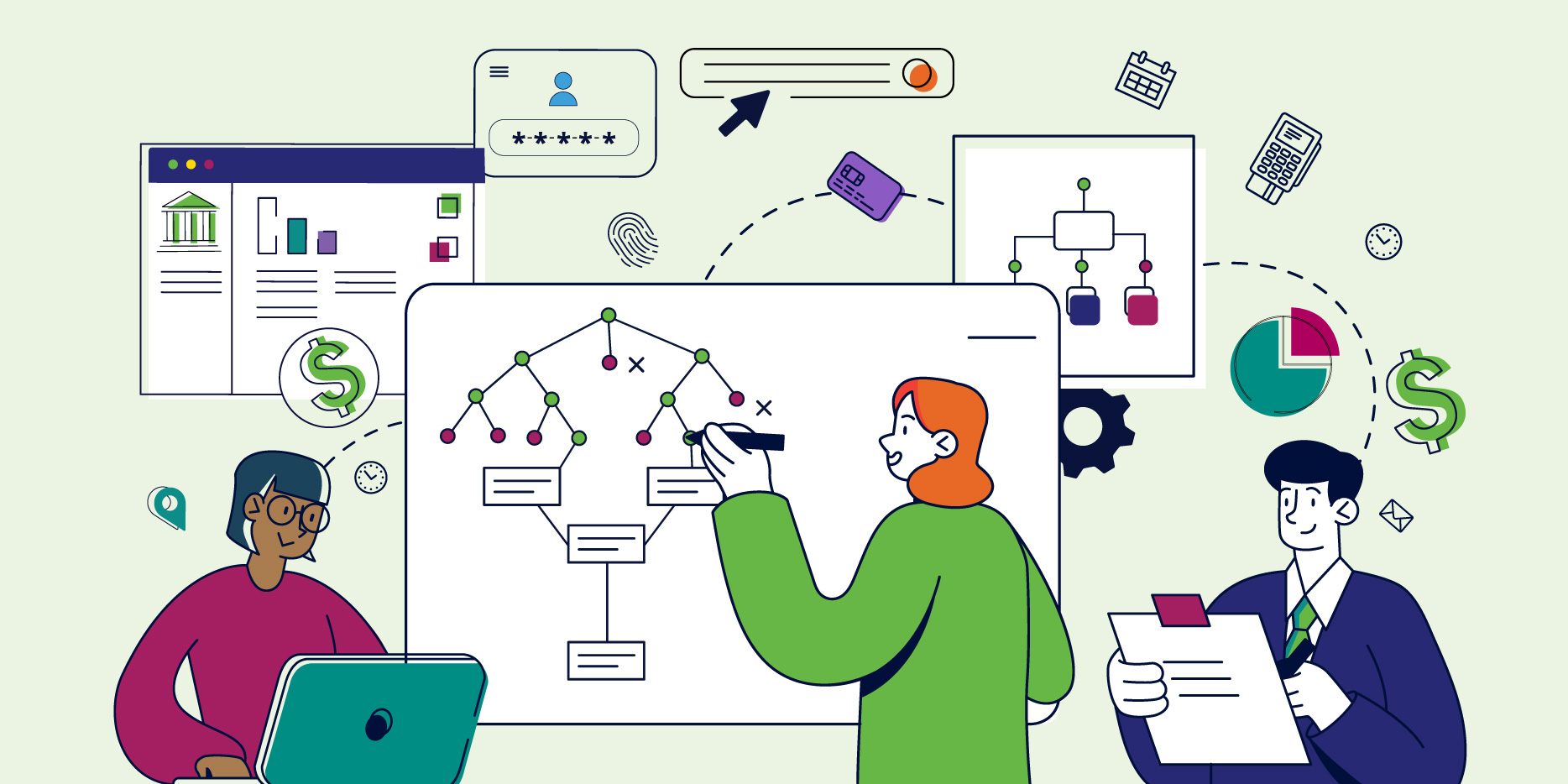
There are numerous machine learning solutions available, and choosing the right one can feel overwhelming for community banks. At the end of the day, however, community banks should ask the following questions about the machine learning solution they want to purchase.
1. Does it aid in the bank’s evolution of becoming a digital-first business?
The solutions should have the ability to score transactions from both old and new digital channels. An even more capable solution will not just score these channels in isolation but will take scores from across channels into consideration when making decisions.
2. Does it assist in the development of new and more modern customer experiences?
With new experiences come major shifts in customer behaviors. Machine learning solutions that are built for community banks should have the capability to adapt to these behavioral changes and, as a result, provide customers with both a secure and smooth banking experience by easily identifying fraud.
3. Does it ensure compliance across banking products?
While doing the above, machine learning solutions need to also ensure that as community banks move through this digital evolution that they don’t unnecessarily expose themselves to risk. Maintaining compliance is at the forefront of every banking official’s mind, and a machine learning solution built for a community bank should not only meet compliance requirements but also help reduce the legwork that goes into it (through reduction of false positives, etc.).
4. Will the vendor do the heavy lifting?
Becoming a machine learning expert doesn’t happen overnight. And, it’s okay if the information doesn’t reside internally. Machine learning providers should have teams that can help guide community banks in the creation of a cohesive data strategy — ensuring that once you make an investment in the technology that you can achieve the maximum benefits.
5. Should I go with a custom or packaged solution?
While custom solutions often boast the most features and overall greatest controllability, they come with their own set of challenges. Custom solutions take a lot of upfront work, often resulting in much longer deployment periods and harder-to-justify ROI at community banking scale. While you might have more options with a custom solution, the higher cost might (and should) quickly disqualify them. Alternatively, packaged, or standardized, solutions are a great way to leverage the benefits of machine learning without incurring nearly the same cost and resulting maintenance requirements.
6. Does the solution deploy on the cloud?
Deploying a solution on the cloud, as compared to on-premise, greatly reduces the overall maintenance costs that are typically associated with machine learning solutions. Also, not having to invest in the framework to support such a solution reduces the necessary upfront investment — and, in turn, the risk of adopting a new ML solution.
Download our eBook, Democratizing Machine Learning for Community Banks for more insights into how community banks can bridge the machine learning gap.
Share this article:
Related Posts
0 Comments6 Minutes
A Guide to Secure, Seamless User Authentication in Payments
Online payments demand a delicate balance between security and user experience. Consumers…
0 Comments7 Minutes
Combating Emerging Scams in the Philippines
The Philippines is witnessing remarkable growth in digital banking. Unfortunately, a…
0 Comments5 Minutes
Feedzai is a Leader in the 2024 IDC MarketScape for Enterprise Fraud Solutions
Exciting news! Feedzai, the world’s first RiskOps platform, is proud to have been named a…